Machine learning (ML) has revolutionized many industries, and retail is no exception. Predictive analytics, powered by machine learning, allows businesses to forecast customer behaviors, optimize inventory management, and improve pricing strategies. By analyzing historical data and detecting patterns, machine learning models can provide actionable insights that lead to improved decision-making and increased profitability.
One of the primary applications of predictive analytics in retail is demand forecasting. Retailers can use machine learning models to predict the demand for specific products based on factors like seasonality, market trends, and past sales data. This helps businesses avoid overstocking or understocking inventory, ensuring they meet customer demand without tying up unnecessary capital in excess goods.
Another significant area where machine learning excels is personalized customer recommendations. By analyzing past purchase behavior, browsing history, and other customer data, retailers can build models that suggest products tailored to individual preferences. This leads to higher conversion rates and customer satisfaction, as shoppers are presented with items they are more likely to buy.
Price optimization is another crucial aspect where machine learning has made a noticeable impact. Dynamic pricing strategies, which adjust prices based on factors such as competitor prices, demand fluctuations, and customer buying behavior, are becoming increasingly popular. Machine learning algorithms can process vast amounts of data in real-time and suggest optimal pricing strategies that maximize profits while maintaining competitive advantage.
Customer segmentation is another powerful application of predictive analytics in retail. By analyzing purchasing behaviors and demographic data, machine learning models can classify customers into distinct groups. This enables retailers to target specific customer segments with personalized marketing campaigns, improving the efficiency and effectiveness of their marketing efforts.
Moreover, machine learning helps retailers identify potential risks, such as supply chain disruptions, fraud, or inventory shrinkage. Predictive models can flag abnormal patterns that indicate fraud or highlight potential issues in the supply chain, allowing businesses to take preventive measures before these problems escalate.
Despite the obvious benefits, implementing machine learning for predictive analytics in retail requires a sound strategy. Retailers must ensure they have access to quality data and the necessary infrastructure to support machine learning models. It’s also important to continuously train and update these models to maintain their accuracy and relevance. The use of machine learning should be seen as a long-term investment, and companies must be prepared to make the necessary investments in technology and expertise.
The future of machine learning in retail looks bright, with advancements in natural language processing (NLP), computer vision, and deep learning enhancing the capabilities of predictive analytics. As more retailers embrace these technologies, the competitive landscape will continue to evolve, with those who successfully integrate machine learning into their operations gaining a significant advantage in the marketplace.
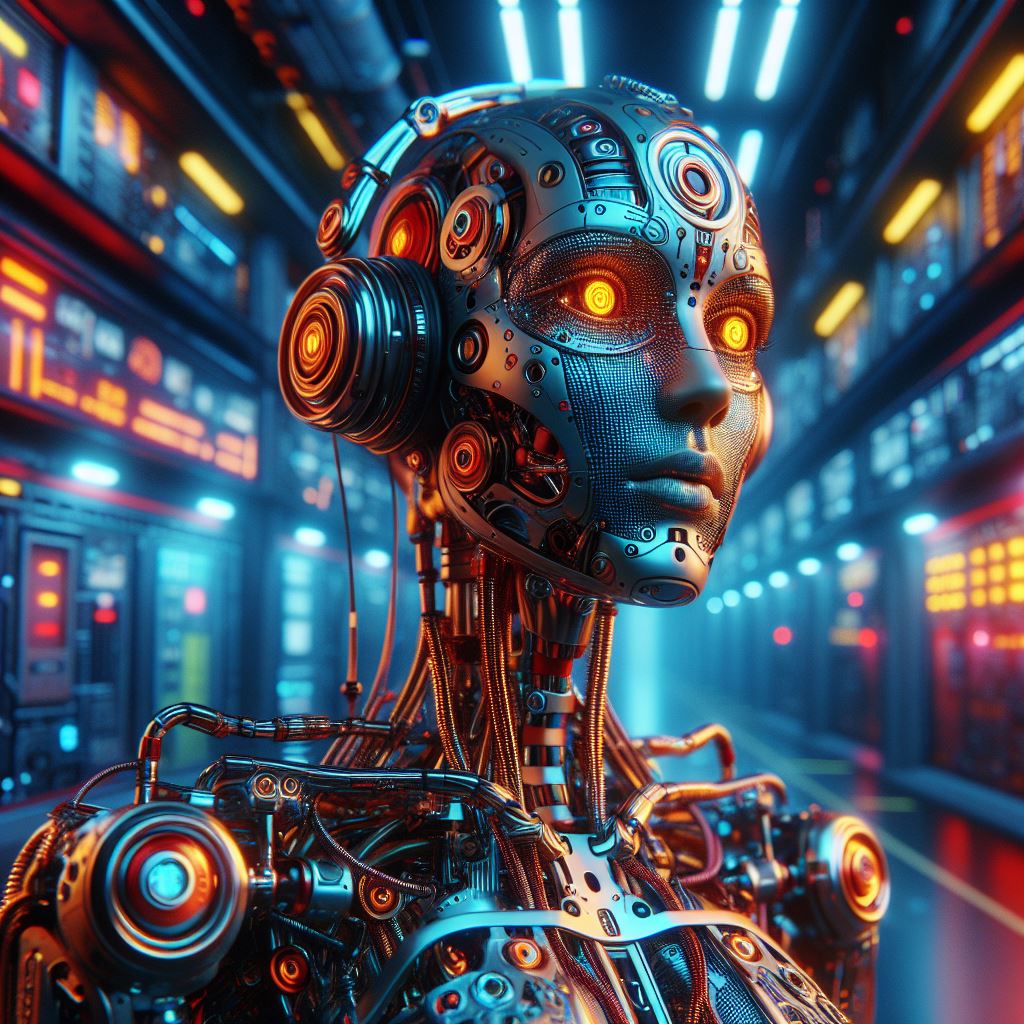