In the world of artificial intelligence (AI), technologies like Retrieval-Augmented Generation (RAG) and Large Language Models (LLMs) are becoming essential for a wide range of applications. These innovations are transforming how machines process and generate language, enabling more efficient and accurate solutions. In this article, we explore how RAG and LLM are revolutionizing the AI landscape, and their potential to impact industries like customer service, content creation, and beyond.
What is Retrieval-Augmented Generation (RAG)?
RAG is an advanced AI framework that combines traditional search-based retrieval with generative language models. The core idea behind RAG is to enhance the output of a language model by providing it with external, relevant information retrieved from a database or documents in real-time. This enables the model to generate responses that are not only contextually accurate but also enriched with up-to-date data, improving both the quality and relevance of its outputs.
By using a retrieval system as part of the generation process, RAG models can handle more complex queries and provide detailed, evidence-backed answers. This approach has become crucial for AI systems that need to operate in domains where knowledge is continuously evolving, such as healthcare, legal services, or technical support.
How Large Language Models (LLMs) Fit Into the RAG Framework
Large Language Models, like OpenAI’s GPT and Google’s BERT, are designed to process and generate human-like text. These models are trained on massive datasets and can handle a variety of tasks, from translation to summarization to question answering. However, LLMs sometimes struggle with producing highly accurate or context-specific answers, especially when they are not equipped with the most current data.
Here’s where RAG becomes valuable. By integrating a retrieval component, RAG-enabled LLMs can access external knowledge sources, dramatically improving their performance on tasks that require real-time information or specialized knowledge. In essence, RAG acts as an augmentation to LLMs, making them more powerful and versatile in real-world applications.
Applications of RAG and LLM in Industry
The combination of RAG and LLM holds immense potential across various sectors. Let’s take a closer look at a few key applications:
Customer Service: AI-driven chatbots and virtual assistants are now a staple of customer support. By leveraging RAG, these systems can provide more accurate and personalized responses, pulling from a company’s knowledge base to give context-specific advice. Whether it’s troubleshooting, product recommendations, or answering FAQs, RAG-enhanced LLMs can elevate the customer experience.
Content Creation: RAG-enabled LLMs are making waves in content generation, from blog posts to marketing copy. By retrieving relevant data from the web or specific sources, these models can create content that is not only well-written but also relevant and informative, ensuring better engagement and SEO performance.
Healthcare: In healthcare, the ability to access and synthesize vast amounts of medical research is crucial. RAG can help AI models provide precise answers to complex medical queries by retrieving the most recent studies, treatments, or diagnoses, making AI a powerful tool for doctors and healthcare professionals.
Legal Services: Law firms can benefit from RAG and LLM by streamlining the process of reviewing legal documents and providing accurate legal advice. By retrieving information from case law databases or client documents, AI systems can offer insights based on the latest legal precedents.
E-commerce: In e-commerce, RAG and LLM can improve product recommendations and support services. AI systems can analyze customer preferences and retrieve relevant product data to provide tailored suggestions, improving conversion rates and overall customer satisfaction.
The Future of RAG and LLM
As AI continues to evolve, we can expect RAG and LLM technologies to become more integrated into daily business operations and consumer applications. With the ongoing advancements in deep learning and retrieval systems, RAG-enabled LLMs will become even more sophisticated, enabling real-time access to vast knowledge networks and delivering highly contextualized results.
The future promises even more breakthroughs in AI-powered customer experiences, productivity tools, and decision-making processes. Companies that embrace RAG and LLM technologies will be better equipped to stay ahead of the competition and offer innovative solutions to their customers.
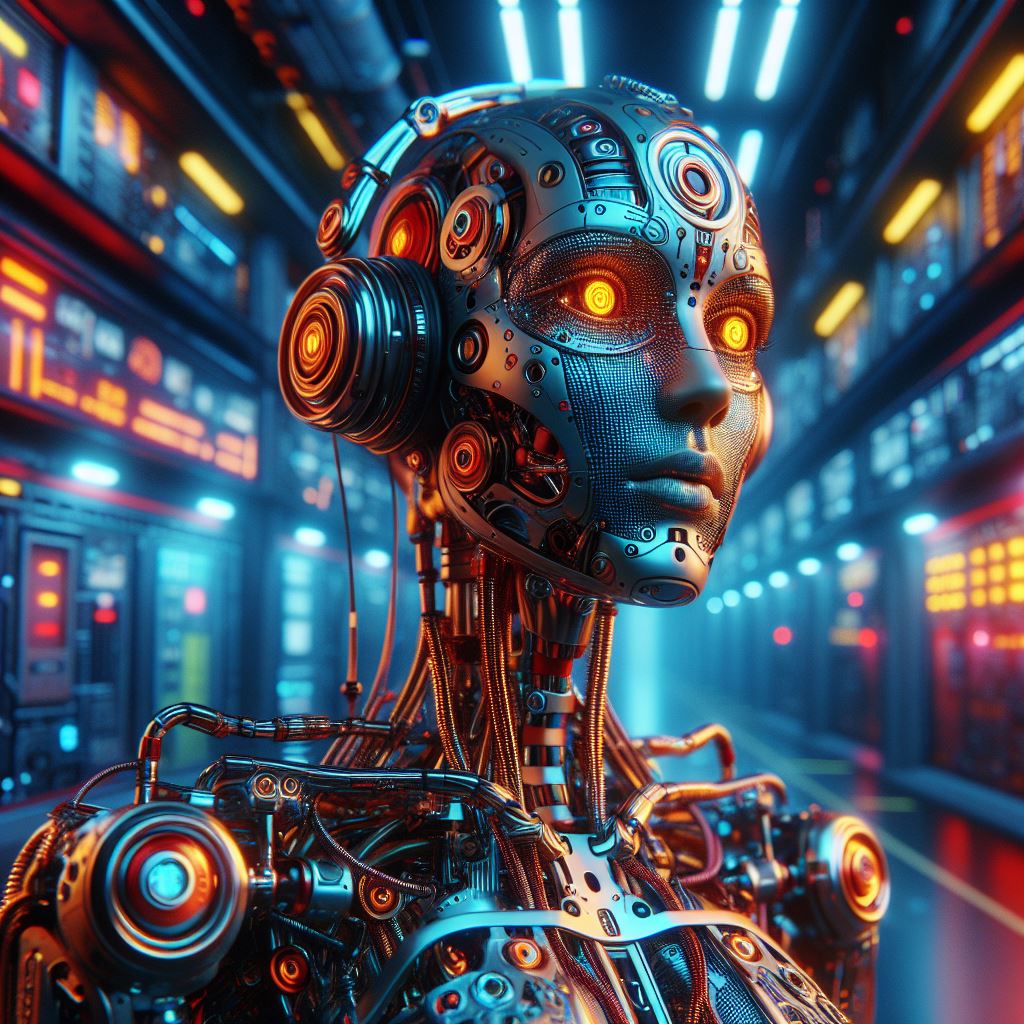