Deep learning, a subset of machine learning, has transformed the landscape of artificial intelligence (AI) over the past decade. With its ability to learn from large datasets and make complex decisions, deep learning has unlocked new possibilities in industries ranging from healthcare to finance. But what exactly is deep learning, and why is it so powerful?
At its core, deep learning mimics the human brain by utilizing neural networks to process information. These networks are composed of layers of interconnected nodes, or “neurons,” which can automatically learn patterns and make predictions. The term “deep” refers to the multiple layers of processing units that enhance the model’s ability to understand and classify data at various levels of abstraction.
One of the key strengths of deep learning is its ability to handle unstructured data, such as images, audio, and text. Traditional machine learning models often struggle with this type of data, requiring extensive feature engineering. In contrast, deep learning models can learn directly from raw data, making them more flexible and efficient. For example, in image recognition tasks, deep learning models can automatically identify objects within images without the need for predefined features.
Deep learning has found applications across many sectors, significantly improving performance in tasks such as computer vision, natural language processing (NLP), and speech recognition. In healthcare, deep learning is used to analyze medical images, helping doctors detect diseases like cancer with higher accuracy. In finance, it is employed for fraud detection, risk assessment, and algorithmic trading, providing more sophisticated insights than traditional methods.
However, despite its impressive capabilities, deep learning does have its challenges. Training deep learning models requires large amounts of labeled data and significant computational resources, which can be costly and time-consuming. Additionally, these models are often seen as “black boxes,” meaning their decision-making process is not always transparent. This lack of interpretability can be a barrier to trust in critical applications, such as autonomous vehicles or medical diagnostics.
To overcome these challenges, researchers are exploring various techniques, such as transfer learning, which allows models to leverage knowledge from pre-trained networks, and explainable AI (XAI), which aims to make deep learning models more transparent and understandable. With continued advancements in hardware, such as specialized processors for AI tasks, and improved algorithms, the future of deep learning looks bright.
In conclusion, deep learning is reshaping the future of AI, enabling machines to perform tasks that were once thought to be exclusive to humans. While it is not without its challenges, the ongoing innovations in deep learning technology hold great promise for a wide range of industries. As we continue to explore the full potential of deep learning, we can expect even more groundbreaking applications in the years to come.
5
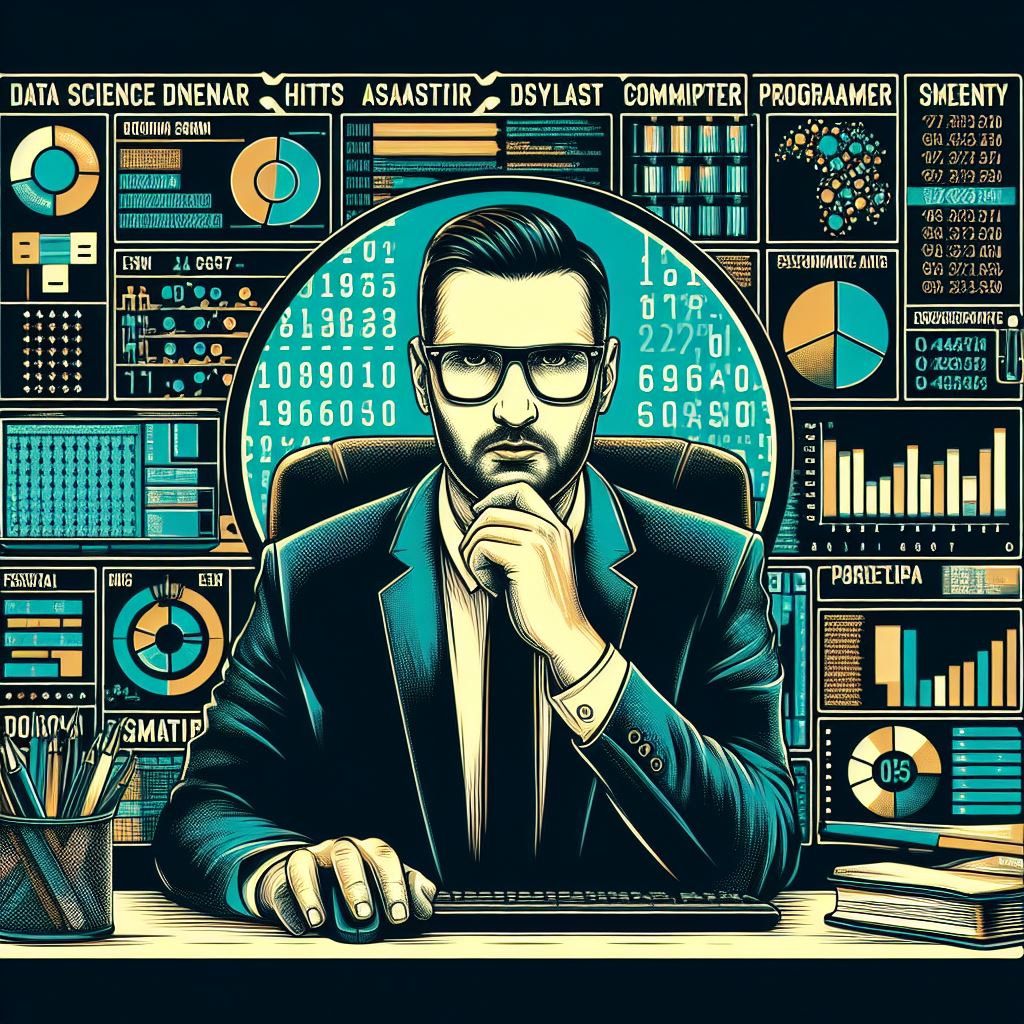