In the ever-evolving world of artificial intelligence (AI), two groundbreaking technologies are shaping the future: Retrieval-Augmented Generation (RAG) and Large Language Models (LLMs). These advancements hold immense potential in transforming how businesses and developers interact with AI systems, enhancing everything from content generation to question-answering systems. Understanding these technologies is crucial for anyone interested in the future of AI-driven solutions.
What is Retrieval-Augmented Generation (RAG)?
Retrieval-Augmented Generation (RAG) is a technique in AI that combines the strengths of both information retrieval and natural language generation. RAG enhances the process of text generation by incorporating external information during the model’s output generation phase. This is accomplished by integrating a retrieval system into the model, allowing it to pull in relevant information from an external knowledge base, such as documents, articles, or databases, before generating a response.
Unlike traditional language models that rely solely on pre-trained knowledge, RAG-based models can access up-to-date and contextually relevant information, leading to more accurate and reliable outputs. This capability makes RAG a powerful tool for tasks such as automated customer support, content creation, and research assistance.
How Do Large Language Models (LLMs) Fit into the Picture?
Large Language Models (LLMs) are a subset of AI models trained on vast amounts of text data, allowing them to generate human-like text based on the input they receive. LLMs, such as GPT-4 and BERT, have revolutionized AI by enabling systems to perform tasks like language translation, summarization, and text generation.
The key feature of LLMs is their ability to generate coherent, contextually relevant content, even when trained on large, diverse datasets. These models work by predicting the next word or phrase in a sequence, making them extremely effective for generating text in real-time. However, while LLMs are powerful, their effectiveness can be limited when they lack access to specific, real-time knowledge.
This is where the combination of RAG and LLMs shines. By combining RAG’s retrieval capabilities with the language generation power of LLMs, AI systems can produce even more accurate and context-aware responses. This synergy allows businesses to build applications that not only generate text but also provide real-time, data-driven insights, making AI more intelligent and versatile.
Applications of RAG and LLMs in Business and Technology
The integration of RAG and LLMs has opened the door to a wide range of applications across industries. One of the most promising areas is in customer service automation. AI-powered chatbots that utilize RAG can pull up the most relevant information from a knowledge base or live databases to assist customers more efficiently. This reduces response times and improves user satisfaction.
Another key application is in content creation. Writers and marketers can leverage RAG-based AI tools to generate high-quality articles, reports, or even marketing materials based on real-time data and external knowledge sources. This ensures that the content is both accurate and tailored to the audience’s needs.
RAG and LLMs are also poised to revolutionize the field of research. By using RAG, AI systems can sift through vast amounts of academic papers, articles, and datasets, retrieving the most relevant information to help researchers solve complex problems faster. This makes the research process more efficient, allowing experts to focus on analysis rather than data collection.
The Future of RAG and LLMs
As the AI landscape continues to evolve, the integration of RAG and LLMs is expected to become even more sophisticated. With improvements in retrieval systems and language models, the accuracy and relevance of AI-generated content will continue to improve. This will lead to more personalized, real-time solutions across a variety of industries, making AI an indispensable tool for businesses and developers alike.
The future holds exciting potential for RAG and LLMs, particularly as they become more widely adopted in everyday applications. Whether it’s enhancing customer service, automating content creation, or advancing research, the combination of retrieval and language models is poised to redefine the role of AI in our lives.
5
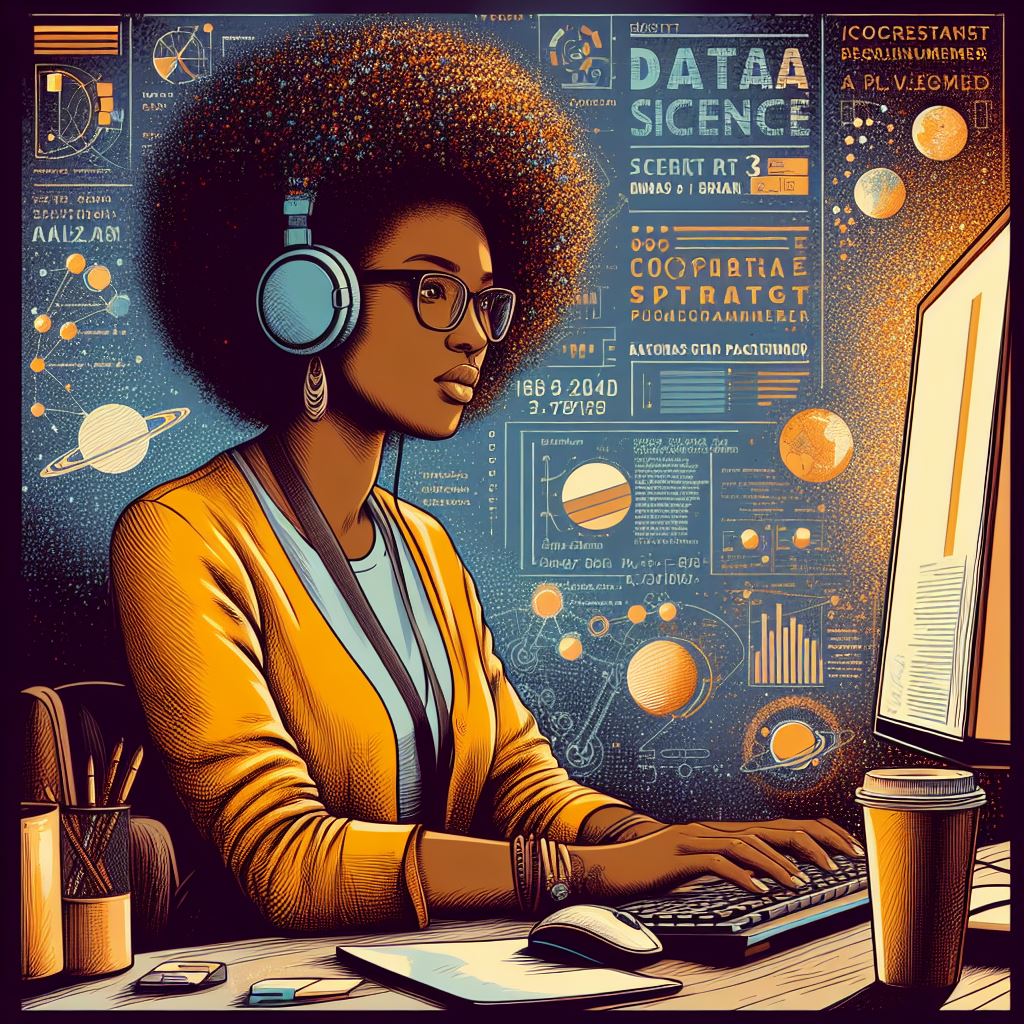