The rapid development of artificial intelligence (AI) has paved the way for innovative solutions across industries. Among the most exciting advancements are Retrieval-Augmented Generation (RAG) and Large Language Models (LLMs), which are reshaping how businesses and organizations process and generate information. In this article, we’ll explore the synergy between RAG and LLMs, their practical applications, and how they are transforming AI-based solutions.
What is RAG?
Retrieval-Augmented Generation (RAG) is a cutting-edge technique that enhances the capabilities of language models by combining retrieval-based approaches with generative models. In a RAG system, the process begins with retrieving relevant information from an external database or knowledge base. This retrieved data is then used to augment the language model’s response, ensuring that the generated output is more accurate, context-aware, and highly informative.
The main advantage of RAG is its ability to blend the strengths of both retrieval and generation. While retrieval ensures that the AI has access to the most up-to-date and relevant data, generation ensures that the AI can deliver coherent, fluent, and creative responses. This combination makes RAG highly effective for applications such as customer service, knowledge management, and personalized recommendations.
How Do Large Language Models (LLMs) Work?
Large Language Models (LLMs) are deep learning models that have been trained on massive datasets to understand and generate human-like text. These models, such as OpenAI’s GPT series, are capable of performing various natural language processing tasks, including text generation, translation, summarization, and question answering. LLMs work by leveraging vast amounts of data to predict the most likely next word in a sequence, allowing them to generate responses that mimic human language patterns.
The scale of these models allows them to understand intricate language nuances, making them ideal for complex tasks like creating content, coding assistance, and interactive conversations. However, LLMs can struggle with keeping responses current or highly accurate if the model was trained on outdated data or lacks domain-specific knowledge.
The Synergy Between RAG and LLM
When combined, RAG and LLMs create a powerful AI framework that enhances the quality and accuracy of generated content. RAG allows LLMs to access relevant, up-to-date information dynamically, mitigating the issues of static knowledge. This synergy is especially valuable in use cases where accurate, real-time responses are critical.
For instance, in a business context, RAG-enhanced LLMs can be used to power customer support chatbots, where they retrieve the latest product data or FAQs to provide accurate and relevant answers. Similarly, in the healthcare industry, RAG and LLMs can assist doctors by pulling recent research papers and medical data to inform their clinical decisions.
Applications of RAG and LLM in Business
Customer Support: With RAG and LLM, businesses can build advanced chatbots and virtual assistants capable of delivering personalized, real-time support. By integrating relevant data into the conversational flow, businesses ensure that customers get accurate and context-aware responses.
Content Creation: Content generation tools powered by RAG and LLMs can help businesses scale their content production, creating blog posts, articles, and social media posts based on the latest industry trends, news, and insights.
Personalized Marketing: AI systems can generate personalized product recommendations by retrieving user behavior data, preferences, and product information. This leads to more accurate targeting in marketing campaigns, driving higher conversion rates.
Healthcare Decision Support: By retrieving medical literature and patient records, RAG and LLMs can provide healthcare professionals with real-time insights, assisting in diagnoses and treatment planning.
Legal and Compliance: In the legal field, RAG and LLMs can be used to retrieve and generate relevant legal documents, case studies, and compliance regulations, aiding lawyers and compliance officers in their work.
Conclusion
The integration of Retrieval-Augmented Generation (RAG) and Large Language Models (LLMs) represents a major leap forward in the development of AI technologies. By combining the power of data retrieval and text generation, businesses can create highly effective AI-driven solutions that are not only accurate but also contextually relevant. As AI continues to evolve, RAG and LLMs will undoubtedly play a pivotal role in shaping the future of business applications, customer interactions, and content creation.
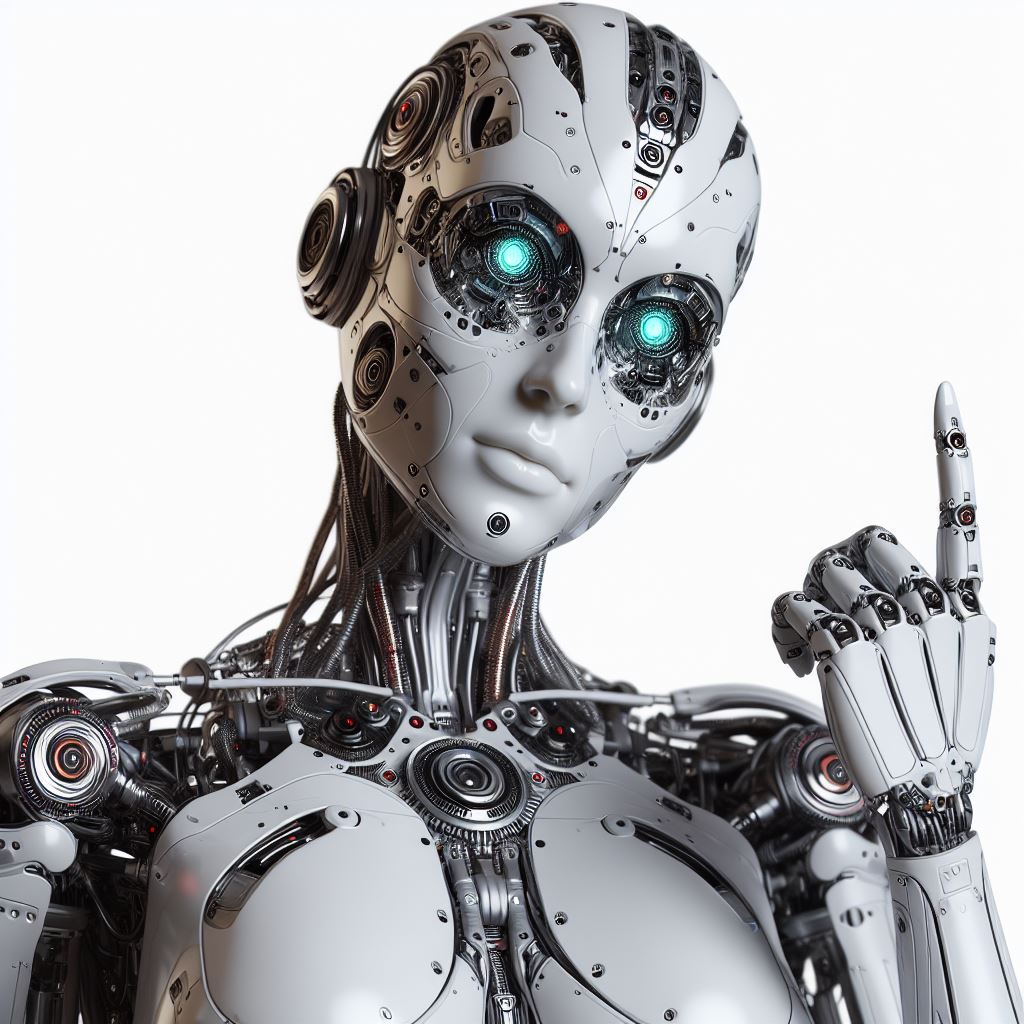