In the rapidly evolving world of artificial intelligence (AI), two key technologies are making waves in enhancing machine learning models and natural language processing: Retrieval-Augmented Generation (RAG) and Large Language Models (LLMs). Both have a profound impact on how AI systems can understand, process, and generate human-like responses, pushing the boundaries of automation, business intelligence, and more. In this article, we’ll dive into the intricacies of RAG and LLM, exploring their differences, use cases, and how they work together to revolutionize the AI landscape.
What is RAG (Retrieval-Augmented Generation)?
Retrieval-Augmented Generation (RAG) is an advanced AI framework that combines the power of information retrieval with text generation. Unlike traditional machine learning models that rely solely on the data they are trained on, RAG allows models to access external information sources to retrieve relevant data before generating a response. This process ensures that the generated answers are not only relevant but also based on up-to-date and accurate information.
RAG has the advantage of improving the knowledge scope of AI models. While traditional models might be limited by their training datasets, RAG models can query external databases, websites, and documents to fetch real-time data, significantly enhancing the accuracy and reliability of the responses. This makes RAG particularly useful for applications such as customer service bots, virtual assistants, and research tools, where the need for both deep understanding and up-to-date information is critical.
How Do Large Language Models (LLMs) Fit In?
Large Language Models (LLMs), such as GPT-3 and GPT-4, are AI systems designed to process and generate human-like text. These models are trained on vast amounts of text data, enabling them to understand language, context, and semantics at an unprecedented level. LLMs use complex algorithms and neural networks to predict the next word or phrase in a sentence based on the input they receive, making them powerful tools for tasks like translation, summarization, and content generation.
The key strength of LLMs lies in their ability to generalize from large datasets, allowing them to generate creative and contextually relevant content. However, they do have limitations, especially when it comes to knowledge that is outside their training data or when they need real-time facts. This is where the integration of RAG comes into play, providing a solution to the static nature of LLMs by enhancing them with retrieval capabilities.
How RAG and LLM Work Together
By combining RAG with LLMs, AI systems can overcome the limitations of both technologies. RAG serves as an external data source that enriches the LLMs’ knowledge by retrieving relevant information, ensuring that the model has access to real-time or specialized data beyond its training set. This hybrid approach leads to better decision-making, more accurate responses, and improved efficiency in various applications.
For instance, in a customer service chatbot powered by RAG and LLM, the system can generate natural, fluent responses (thanks to the LLM) while simultaneously pulling in relevant product details, FAQ entries, or troubleshooting guides from external sources (thanks to RAG). This combination allows the AI to handle more complex queries, providing more comprehensive and contextually appropriate answers.
Applications of RAG and LLM in Business
RAG and LLM are paving the way for more intelligent and responsive AI-driven applications across various industries. In e-commerce, businesses can use these technologies to create advanced virtual assistants that provide personalized recommendations and real-time customer support. In healthcare, RAG and LLM can help process vast amounts of medical data, offering insights and generating reports based on the latest research.
Furthermore, these technologies can transform content creation by helping generate high-quality articles, reports, and marketing materials quickly and efficiently, reducing the time and effort required for human writers. In the field of education, they can assist in personalized learning, providing students with targeted information and resources based on their individual needs and learning progress.
Conclusion
RAG and LLM are powerful technologies that, when combined, offer the potential to transform how AI systems interact with and process information. By enabling AI models to retrieve up-to-date data and generate contextually relevant content, RAG and LLM are revolutionizing industries and setting the stage for even more advanced AI applications in the future. As these technologies continue to evolve, we can expect even more sophisticated and accurate AI-driven solutions across a wide range of sectors.
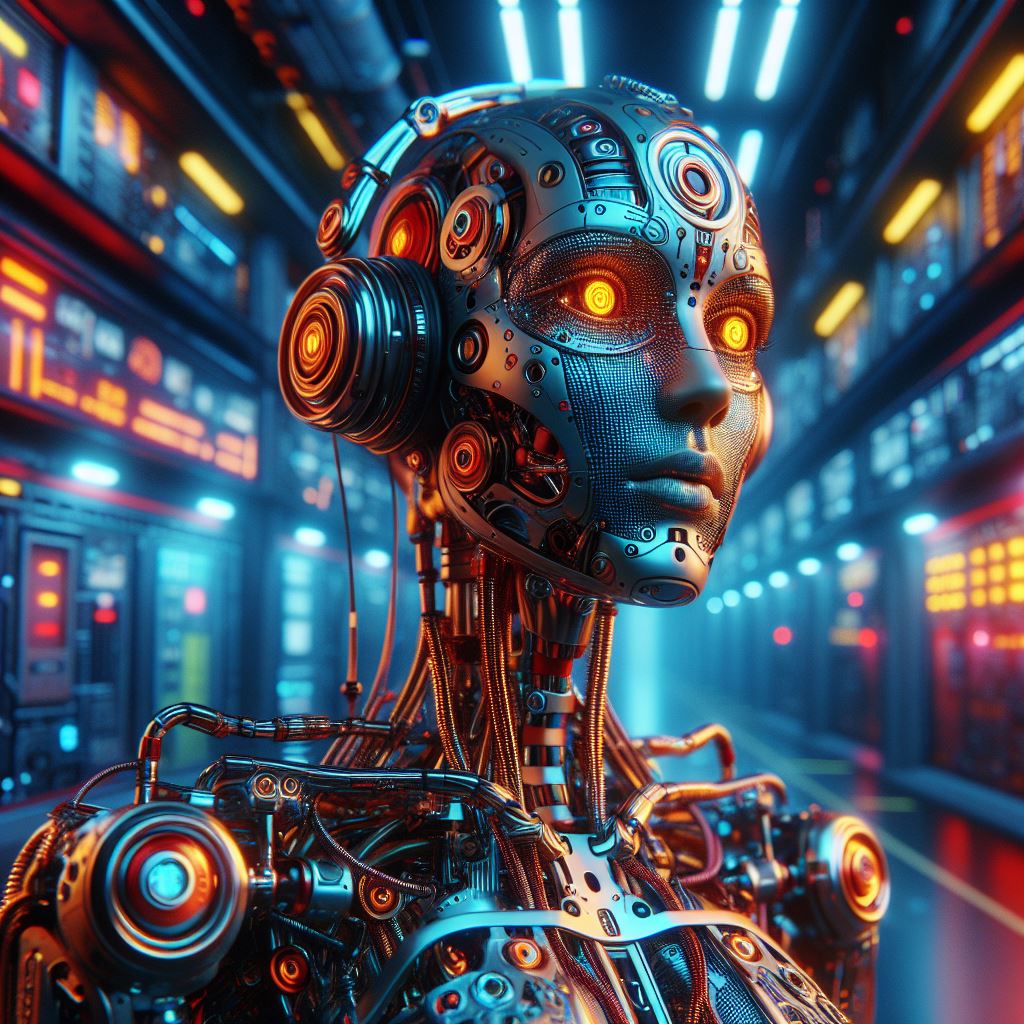