In recent years, the field of Artificial Intelligence (AI) has witnessed significant breakthroughs, with technologies like Retrieval-Augmented Generation (RAG) and Large Language Models (LLMs) taking center stage. These innovations are transforming how businesses interact with data, offering powerful tools to solve complex challenges in real-time. This article explores the concepts of RAG and LLMs, their importance, and how they can be leveraged by organizations to optimize processes and improve decision-making.
What is RAG (Retrieval-Augmented Generation)?
Retrieval-Augmented Generation (RAG) is an advanced AI technique designed to enhance the performance of language models by retrieving relevant information from external sources before generating responses. Unlike traditional models that rely solely on pre-trained data, RAG integrates a retrieval mechanism that allows it to fetch up-to-date and contextually relevant information, improving the quality and accuracy of its output.
This technology is particularly valuable in scenarios where the AI model needs to answer specific queries based on documents, databases, or other external sources. By combining the power of document retrieval and language generation, RAG can deliver more accurate and tailored responses, making it an essential tool for businesses seeking to provide precise, real-time answers to customer inquiries or internal analyses.
What are LLMs (Large Language Models)?
Large Language Models (LLMs) are a class of AI models trained on vast amounts of text data. They are designed to understand, generate, and manipulate human language in a way that mimics human communication. With the ability to process and generate text based on patterns found in large datasets, LLMs are capable of performing a wide range of tasks, from answering questions to creating coherent articles, translating languages, and even generating creative content.
LLMs like GPT-3 and GPT-4, developed by OpenAI, have demonstrated exceptional capabilities in natural language understanding and generation. These models are not just powerful tools for text generation but can also be integrated into various business solutions, such as customer support, content creation, and data analysis, making them indispensable assets for modern businesses.
How RAG and LLMs Work Together
When combined, RAG and LLMs offer a powerful solution for businesses looking to leverage AI for information retrieval and content generation. The RAG framework enhances the performance of LLMs by providing real-time access to relevant documents and data sources, which allows the language model to generate responses that are more contextually accurate and informative.
For instance, in a customer support scenario, a business could use RAG to retrieve the most relevant information from a knowledge base or customer database, while the LLM generates a human-like response based on this information. This combination enables businesses to provide faster, more accurate support to customers, improving satisfaction and reducing response times.
Applications of RAG and LLMs in Business
Customer Support: By integrating RAG with LLMs, companies can build intelligent customer service bots that provide quick and accurate responses by pulling information from knowledge bases, FAQs, and customer data.
Content Generation: Businesses in content-heavy industries, such as marketing and media, can use LLMs to generate articles, blog posts, and reports, while RAG ensures that the content is based on the most up-to-date and relevant information available.
Data Analysis: RAG-powered LLMs can assist in analyzing large datasets and extracting meaningful insights, which is crucial for decision-making in fields like finance, healthcare, and e-commerce.
Knowledge Management: Organizations can use RAG with LLMs to create more effective knowledge management systems, allowing employees to quickly access the information they need from vast document repositories.
Personalization: RAG can enhance personalization efforts by retrieving tailored information based on user preferences and historical data, while LLMs can use this data to generate customized recommendations and responses.
Conclusion
RAG and LLMs are reshaping the future of AI by enabling businesses to access and generate precise, contextually relevant information in real-time. Their integration holds the potential to streamline operations, improve customer experiences, and drive smarter decision-making. As these technologies continue to evolve, they are bound to become even more integral to business strategies across various industries.
5
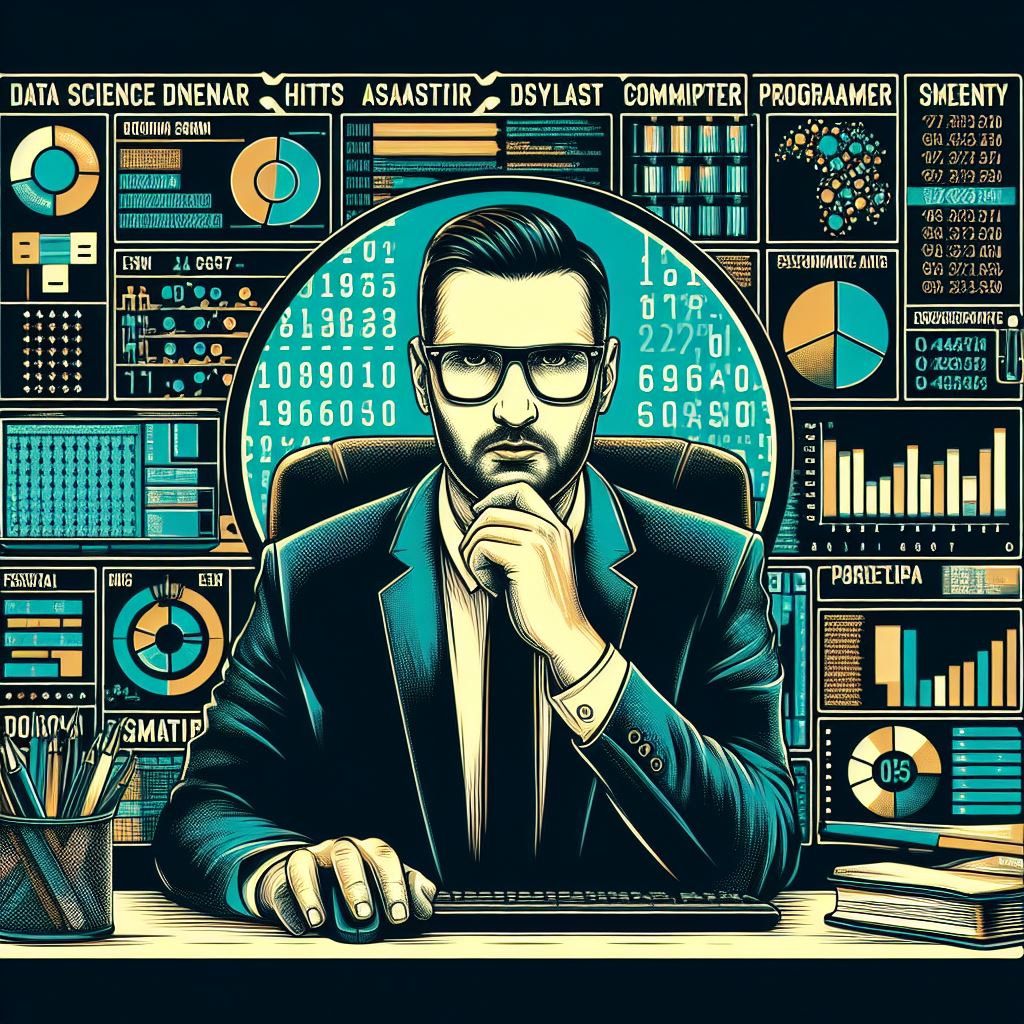