Few-shot learning is a revolutionary concept in the field of machine learning and artificial intelligence. It refers to the ability of a machine learning model to learn effectively from only a few examples, as opposed to traditional methods that require large datasets. This innovative approach is gaining immense popularity due to its potential to reduce the need for vast amounts of labeled data, making it particularly valuable in real-world applications where data is scarce or difficult to obtain.
In traditional machine learning, algorithms rely heavily on large labeled datasets to train models. This process, while effective, is resource-intensive and time-consuming. Few-shot learning, however, aims to address these challenges by enabling models to generalize from a limited number of examples, mimicking human learning processes. For instance, humans can recognize objects, identify faces, or understand concepts after seeing only a few examples. Few-shot learning attempts to replicate this remarkable human capability in machines.
One of the key techniques used in few-shot learning is transfer learning, which leverages pre-trained models on large datasets and fine-tunes them for specific tasks with only a few labeled examples. This approach is especially useful in domains like natural language processing (NLP), computer vision, and speech recognition. Transfer learning allows models to apply knowledge gained from one domain to another, accelerating learning and improving performance even with limited data.
Another important method in few-shot learning is meta-learning, which focuses on training models to learn how to learn. In meta-learning, a model is trained across various tasks to develop a strategy for learning new tasks with minimal data. This enables models to adapt quickly to new scenarios without needing extensive retraining. Meta-learning has proven to be highly effective in fields like robotics and autonomous systems, where rapid adaptation to new environments is critical.
Applications of few-shot learning are wide-ranging. In computer vision, few-shot learning can be used to recognize new objects or identify rare diseases from medical images with limited annotated data. In natural language processing, it enables machines to understand new languages or dialects with only a few sentences. Additionally, in speech recognition, few-shot learning helps systems recognize new accents or speech patterns with minimal examples.
Despite its impressive capabilities, few-shot learning still faces several challenges. One of the main hurdles is ensuring that the model generalizes well to new tasks without overfitting to the limited data it has seen. Moreover, the quality of the few examples provided is crucial—if they are not representative of the task at hand, the model’s performance can degrade significantly.
In conclusion, few-shot learning is a game-changer in machine learning, offering the ability to build powerful models with minimal data. By combining techniques like transfer learning and meta-learning, this approach is unlocking new possibilities for artificial intelligence in fields where data is limited or costly. As research in this area progresses, we can expect even more advanced solutions that will revolutionize industries from healthcare to entertainment.
5
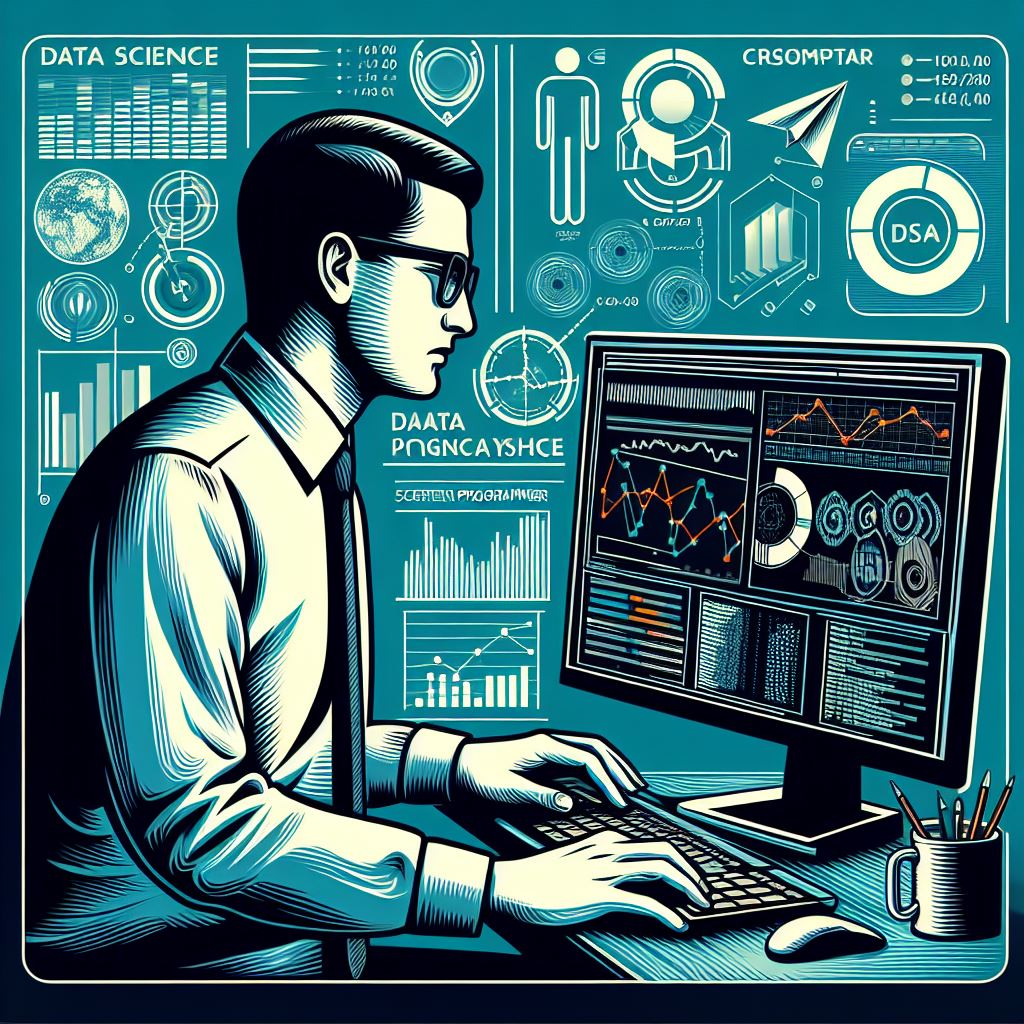