Deep learning is a subset of machine learning that has revolutionized the field of artificial intelligence (AI) by mimicking the human brain’s architecture to process and analyze vast amounts of data. As a branch of AI, deep learning uses neural networks with many layers to extract features from data, enabling the machine to make intelligent decisions. This technology powers many applications, from speech recognition and natural language processing (NLP) to autonomous vehicles and healthcare.
The concept of deep learning is rooted in neural networks, which were inspired by the way neurons in the human brain work. These networks consist of multiple layers of interconnected nodes, called artificial neurons. Each layer processes information and passes it to the next layer, creating a complex system capable of learning from large datasets. This layered architecture allows deep learning algorithms to recognize patterns and make predictions with exceptional accuracy.
One of the most significant advantages of deep learning over traditional machine learning algorithms is its ability to handle unstructured data. For example, images, audio, and text, which were previously difficult for computers to process, can now be understood and analyzed by deep learning models. Convolutional neural networks (CNNs) and recurrent neural networks (RNNs) are two of the most commonly used deep learning architectures. CNNs are particularly effective in image processing, while RNNs excel at sequential data like time series or text.
The application of deep learning is seen in everyday technology. In healthcare, deep learning models can analyze medical images to identify diseases such as cancer, offering early detection and improving treatment outcomes. In the automotive industry, autonomous driving systems rely heavily on deep learning to understand and navigate the world around them. Additionally, deep learning has transformed natural language processing, powering virtual assistants like Siri and chatbots that provide real-time, accurate responses to users.
Despite its potential, deep learning also has its challenges. Training deep learning models requires vast amounts of data and significant computational power. The process of training a model involves adjusting millions or even billions of parameters to optimize performance, which can be time-consuming and expensive. Moreover, deep learning models are often seen as “black boxes,” meaning their decision-making process is not always transparent, which can lead to issues with accountability and trust.
Another challenge is the need for large labeled datasets to train deep learning models. Gathering such data can be time-consuming, expensive, and sometimes difficult, especially for specialized applications where labeled data is scarce. Additionally, deep learning models require a significant amount of energy for training and deployment, which raises concerns about their environmental impact.
Despite these challenges, deep learning continues to be at the forefront of AI research and development. The technology is constantly evolving, with new architectures and algorithms being developed to improve efficiency and accuracy. Researchers are working on creating more explainable deep learning models, and advancements in hardware, such as specialized processors like GPUs and TPUs, are helping to make deep learning more accessible and scalable.
As deep learning becomes more advanced, it has the potential to transform industries and improve lives in ways we can only begin to imagine. From healthcare and finance to entertainment and transportation, the future of AI is deeply intertwined with deep learning. As we continue to develop and refine this technology, the possibilities for innovation and growth seem limitless.
5
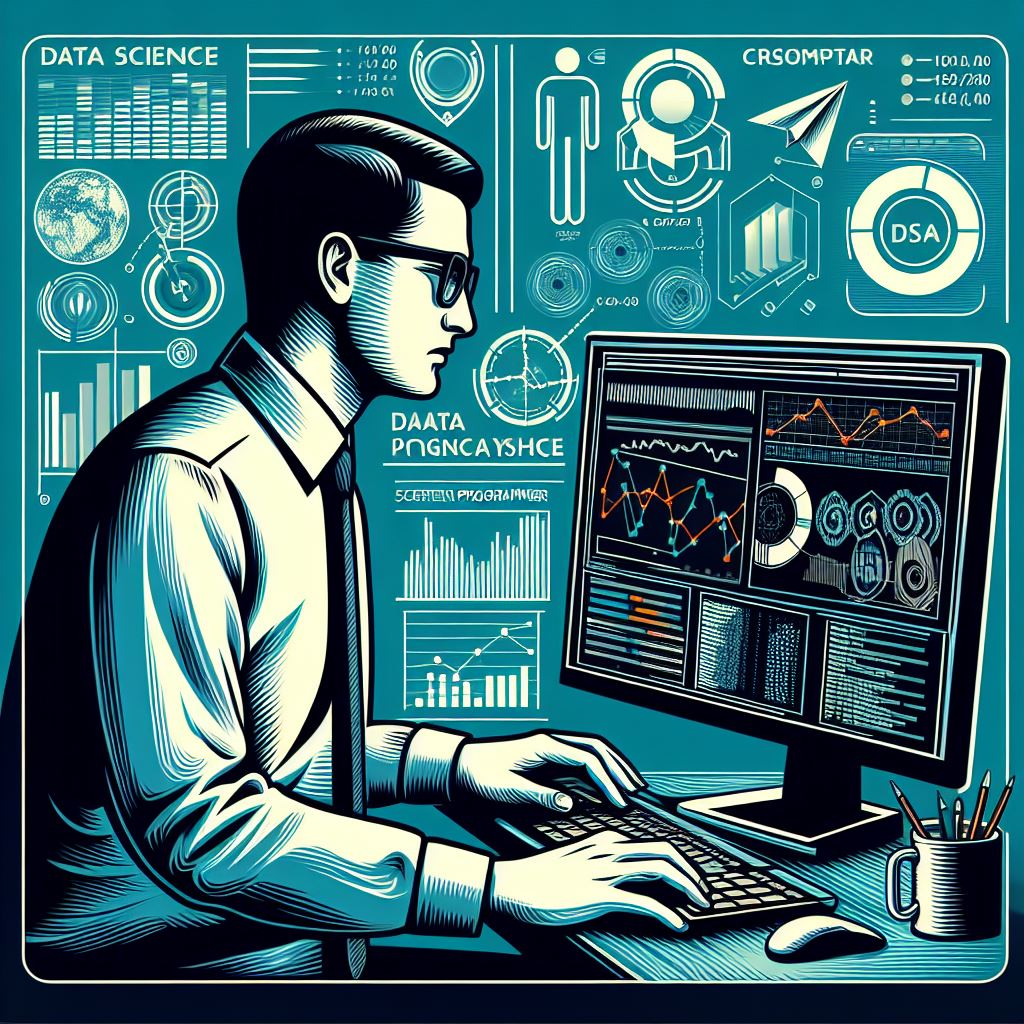