Deep learning is one of the most innovative advancements in the field of artificial intelligence (AI). It has revolutionized how machines learn from data, allowing them to perform tasks that were once considered impossible for computers. Deep learning algorithms are capable of recognizing patterns, understanding complex structures, and making decisions based on large amounts of data. This technology mimics the way the human brain processes information, using a structure known as artificial neural networks (ANNs).
At its core, deep learning involves training neural networks with many layers, allowing the system to learn from data in a hierarchical manner. Each layer of the network extracts different features from the input data, progressively refining the results. The ability to process vast amounts of unstructured data, such as images, text, and audio, has made deep learning a powerful tool in many industries, including healthcare, finance, automotive, and entertainment.
Key Concepts in Deep Learning
Neural Networks
Neural networks are the building blocks of deep learning. These networks consist of layers of interconnected nodes, or artificial neurons, which process input data. Each node performs a mathematical operation, passing the result to the next layer in the network. This network of nodes is trained to learn patterns from the input data and improve its performance over time.
Training and Optimization
Deep learning models are trained by feeding them large datasets and adjusting the weights of the connections between neurons. The goal is to minimize the difference between the predicted output and the actual result, a process known as “backpropagation.” Over time, the network becomes more accurate in its predictions by refining its internal parameters.
Convolutional Neural Networks (CNNs)
CNNs are a specialized type of neural network commonly used for image recognition tasks. These networks use convolutional layers to detect patterns, such as edges, shapes, and textures, in images. By progressively combining these patterns, CNNs can identify objects and scenes in photos and videos with high accuracy.
Recurrent Neural Networks (RNNs)
RNNs are designed for processing sequential data, such as time-series data or natural language. Unlike traditional neural networks, RNNs have connections that allow information to persist over time, making them ideal for tasks like speech recognition, language translation, and predicting stock prices.
Generative Models
Deep learning also includes generative models like Generative Adversarial Networks (GANs), which are capable of generating new, realistic data. GANs consist of two networks: a generator, which creates data, and a discriminator, which evaluates it. This dynamic competition helps improve the generated data, making it nearly indistinguishable from real-world data.
Applications of Deep Learning
Deep learning has transformed several industries, making it possible to automate tasks that once required human intelligence. In healthcare, deep learning algorithms can analyze medical images and predict disease outcomes, helping doctors diagnose patients more accurately. In the automotive industry, deep learning is used in self-driving cars to recognize objects and make driving decisions in real-time.
In the entertainment industry, platforms like Netflix and Spotify use deep learning to recommend content based on users’ preferences. Additionally, deep learning is a driving force behind advancements in natural language processing (NLP), powering virtual assistants like Siri, Alexa, and Google Assistant, which can understand and respond to voice commands.
Future of Deep Learning
The potential for deep learning is limitless. As computational power continues to increase and datasets become even larger and more diverse, deep learning models will become more powerful and efficient. Future developments may lead to breakthroughs in AI applications that we cannot yet predict, opening new doors for innovation across various sectors.
Conclusion
Deep learning is an exciting and transformative technology that is changing the landscape of artificial intelligence. From healthcare to entertainment, its applications are vast and growing. As this field continues to evolve, deep learning will play an increasingly critical role in shaping the future of AI and automation.
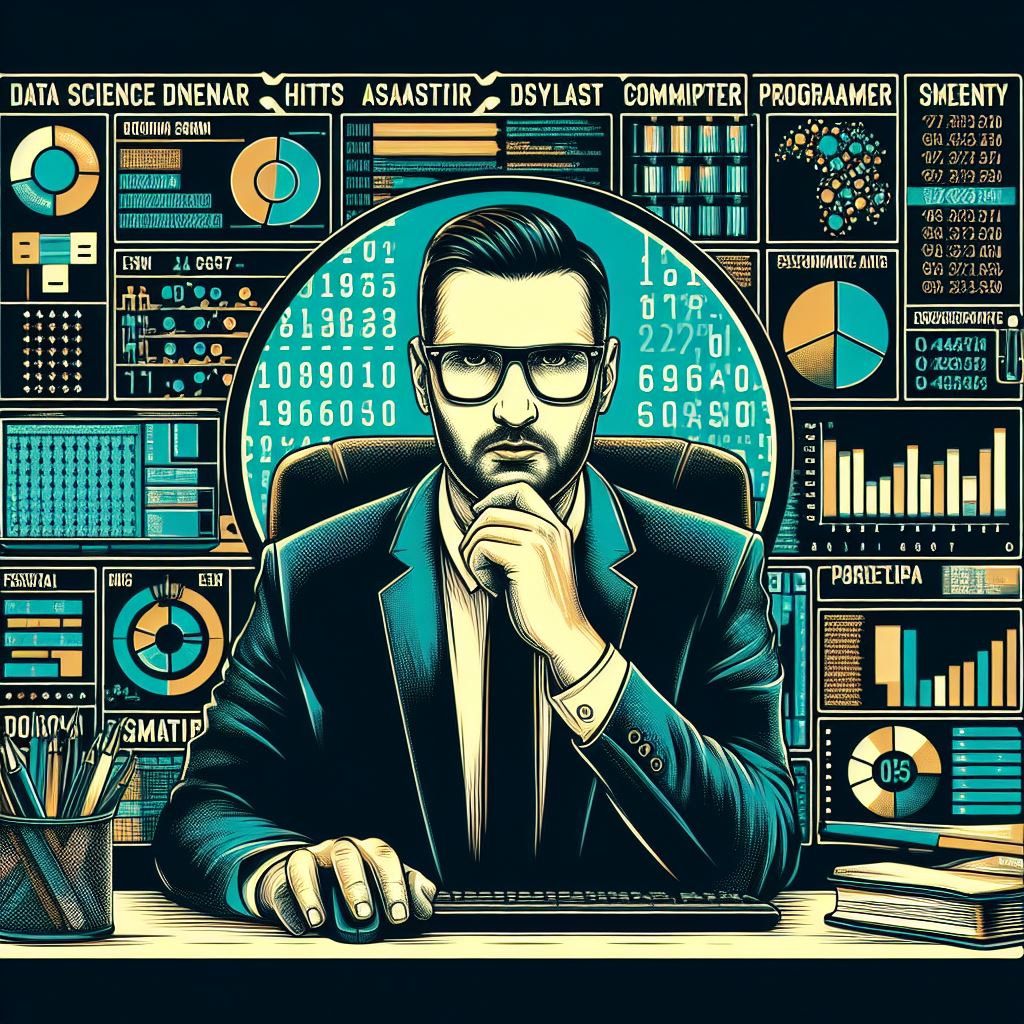