Deep learning is a subfield of machine learning that has revolutionized the way we approach artificial intelligence (AI) problems. It is based on algorithms inspired by the structure and function of the human brain, known as artificial neural networks. This technology enables computers to learn from vast amounts of data, making it possible to perform tasks such as image recognition, natural language processing, and self-driving cars with remarkable accuracy.
At the heart of deep learning is the concept of neural networks. These networks consist of layers of interconnected nodes or “neurons,” which mimic the way biological neurons in the brain transmit information. A deep neural network has many layers, hence the term “deep” learning. Each layer progressively extracts features from the input data, allowing the system to make complex decisions based on that data.
One of the most significant advantages of deep learning is its ability to automatically extract features from raw data, eliminating the need for manual feature engineering. For example, in image recognition tasks, traditional machine learning models would require a human to manually select features such as edges or shapes. In contrast, deep learning models can learn these features directly from the data, making them more efficient and accurate.
Deep learning is also highly scalable. With the increasing availability of large datasets and powerful computing resources, deep learning models can be trained on massive amounts of data, allowing them to improve over time. This scalability has led to breakthroughs in various fields, such as healthcare, finance, and autonomous driving. In healthcare, for instance, deep learning models are being used to detect diseases like cancer from medical images, while in finance, they are used for fraud detection and risk assessment.
However, deep learning does come with its challenges. One of the biggest hurdles is the need for large amounts of labeled data. Training a deep learning model requires a substantial amount of high-quality, labeled data, which can be difficult and expensive to obtain. Additionally, deep learning models are computationally intensive, requiring powerful hardware such as Graphics Processing Units (GPUs) to train effectively. Despite these challenges, the results achieved by deep learning models in real-world applications have made it one of the most exciting areas of research in AI today.
Another area where deep learning is making an impact is natural language processing (NLP). With the advent of deep learning models like transformers, machines can now understand and generate human language with unprecedented accuracy. These models are used in applications such as chatbots, machine translation, and sentiment analysis. The ability of deep learning models to process and interpret text has brought about significant advancements in customer service, content creation, and information retrieval.
The future of deep learning is bright. As research progresses, we can expect even more sophisticated models capable of solving increasingly complex problems. Advances in explainability, model interpretability, and efficiency will likely make deep learning even more accessible and widely used across different industries.
In conclusion, deep learning has emerged as a game-changing technology that continues to reshape the landscape of artificial intelligence. By mimicking the human brain’s neural networks, it allows computers to learn from data and perform tasks that were once thought impossible. With its scalability, ability to learn from raw data, and applications across a variety of fields, deep learning is set to remain at the forefront of AI development for years to come.
5
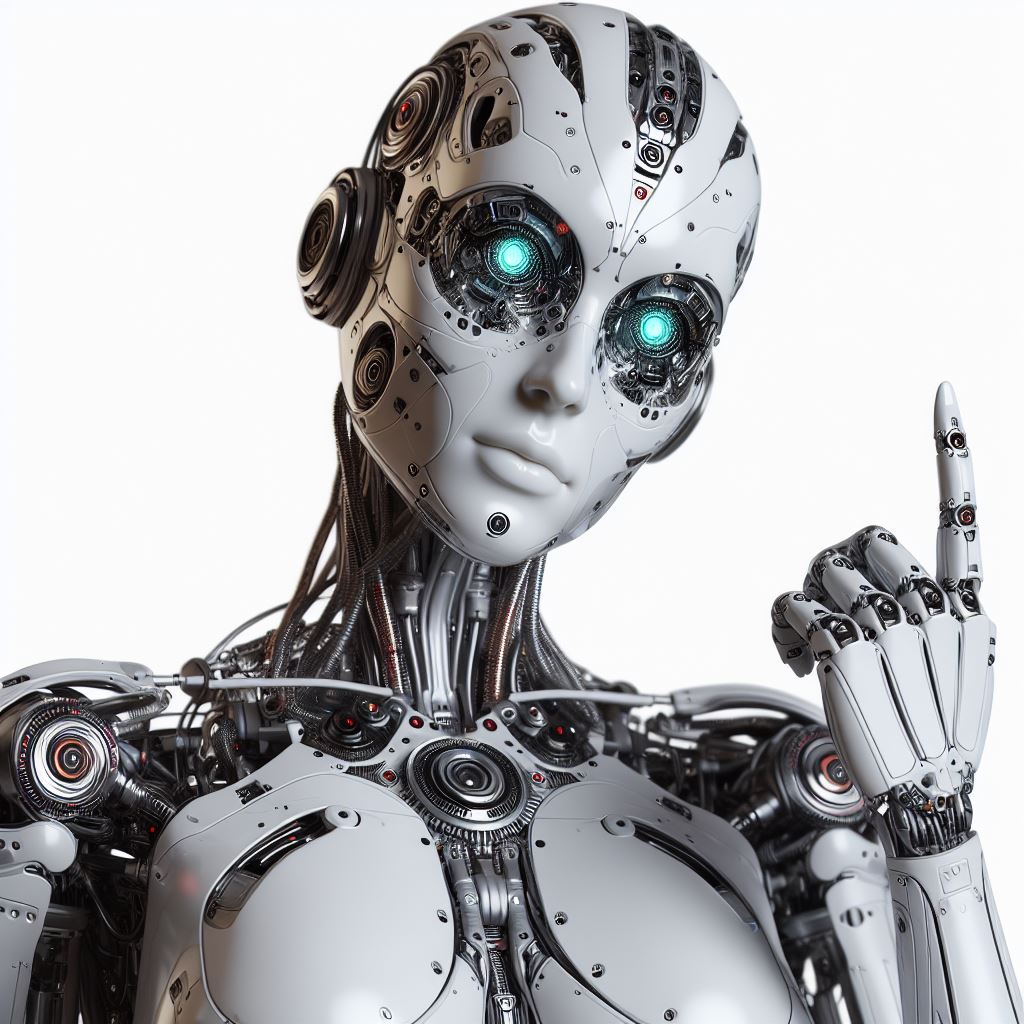