Deep learning is a subset of artificial intelligence (AI) that has revolutionized the way machines understand and process data. With its ability to automatically learn from vast amounts of data, deep learning is reshaping various industries, from healthcare to finance. In this article, we will explore what deep learning is, its applications, and why it’s a game changer for the future.
Deep learning is inspired by the structure of the human brain and involves neural networks with many layers. These deep neural networks (DNNs) are designed to identify patterns in large datasets, enabling machines to make decisions, recognize objects, translate languages, and even play complex games at superhuman levels.
The key strength of deep learning lies in its ability to handle unstructured data, such as images, audio, and text. Traditional machine learning algorithms struggle with this kind of data, but deep learning networks excel by learning directly from raw data without the need for manual feature extraction. For instance, in image recognition, deep learning models can identify objects, faces, and scenes with remarkable accuracy, making them indispensable in fields like security, healthcare, and autonomous driving.
In healthcare, deep learning is transforming diagnostics and medical imaging. AI-driven systems can analyze medical scans such as X-rays, CT scans, and MRIs to detect anomalies, such as tumors, with high precision. This capability not only accelerates diagnosis but also helps doctors provide better treatment plans. Similarly, deep learning algorithms are used to predict patient outcomes, personalize treatments, and discover new drugs.
The finance industry is also benefiting from deep learning through enhanced fraud detection, algorithmic trading, and risk management. By analyzing historical transaction data and market trends, deep learning models can identify fraudulent activities in real-time and predict market movements, helping investors make more informed decisions.
Another exciting application of deep learning is in natural language processing (NLP), which is used to improve chatbots, translation services, and sentiment analysis. Deep learning models like GPT-3 (the technology behind many AI writing assistants) can generate human-like text, answer questions, and even hold conversations, demonstrating the power of AI in language understanding and generation.
Despite its remarkable achievements, deep learning is not without its challenges. The need for massive amounts of labeled data and computational resources can be a barrier for some businesses. Training deep learning models requires powerful GPUs, extensive data storage, and energy consumption, which can make the technology costly to implement. Additionally, deep learning models are often viewed as “black boxes,” meaning their decision-making process is not always transparent, making it harder to trust in high-stakes applications.
Nevertheless, deep learning continues to evolve, with researchers developing more efficient models and exploring ways to overcome these obstacles. The advent of transfer learning, for example, allows pre-trained models to be fine-tuned for specific tasks with less data, making deep learning more accessible and cost-effective.
In conclusion, deep learning is one of the most exciting advancements in the field of AI, offering transformative possibilities across various industries. From improving healthcare diagnostics to optimizing financial services, the applications of deep learning are vast and growing. As the technology continues to evolve, it promises to unlock even more innovations, paving the way for smarter machines and a more connected world.
5
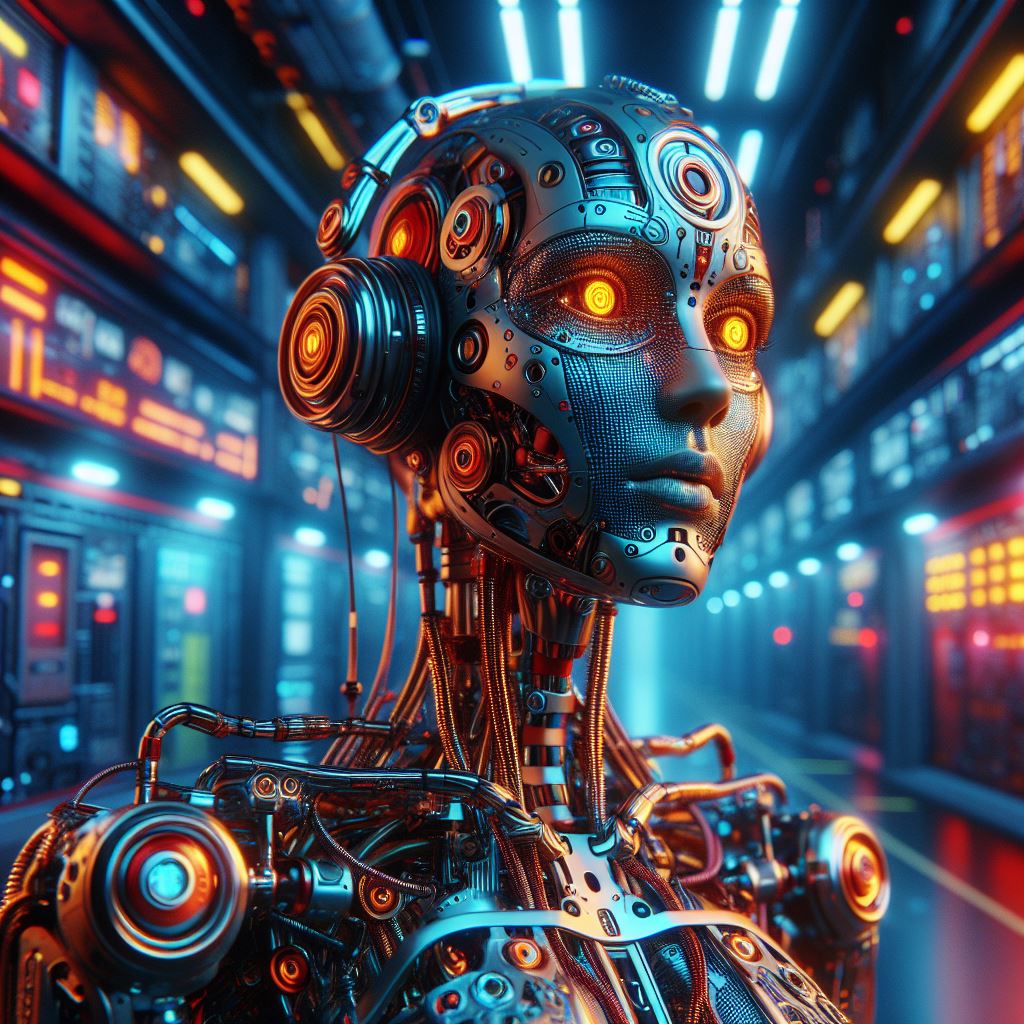