In recent years, reinforcement learning (RL) has emerged as a groundbreaking technology in the field of artificial intelligence (AI), showing immense promise in revolutionizing various industries. One area where RL is making significant strides is in robotics for agriculture. This combination of advanced AI techniques and robotics is poised to transform how farms operate, increase productivity, and reduce environmental impact. Here, we explore the potential of reinforcement learning in robotics and its impact on the future of agriculture.
What is Reinforcement Learning?
Reinforcement learning is a type of machine learning where an agent learns how to make decisions by performing actions in an environment to achieve a goal. The agent receives feedback in the form of rewards or penalties based on its actions. Over time, the agent learns the best strategies to maximize rewards and minimize penalties, essentially teaching itself how to make optimal decisions. In the context of robotics, RL allows robots to learn tasks without explicit programming, making them adaptable to complex and dynamic environments.
Applications of RL in Robotics for Agriculture
Robotic systems, powered by reinforcement learning, are being increasingly deployed in agricultural settings for a variety of tasks. These robots can navigate fields, interact with crops, and perform specific operations like harvesting, planting, and monitoring. Here are some key applications:
1. Autonomous Harvesting:
Reinforcement learning can be used to train robots to identify ripe crops and harvest them efficiently. These robots use sensors and cameras to assess the maturity of crops and determine the optimal time for picking. By learning from previous actions, they become more proficient over time, reducing waste and improving harvest yields.
2. Precision Agriculture:
Precision agriculture involves using technology to monitor and optimize crop production. RL-powered robots can analyze soil conditions, moisture levels, and pest infestations, making real-time adjustments to irrigation and fertilization. This leads to more efficient resource use, increased crop yield, and reduced environmental impact.
3. Weed Control:
Robotic systems equipped with RL algorithms can identify and eliminate weeds with precision. Rather than spraying chemicals over large areas, these robots target individual weeds, reducing the need for pesticides and minimizing harm to surrounding plants. This approach is not only more sustainable but also enhances crop health and yields.
4. Planting and Seeding:
Reinforcement learning enables robots to learn the best planting patterns based on environmental variables and crop types. By optimizing planting depth, spacing, and timing, RL robots contribute to better crop establishment and overall field productivity.
5. Data Collection and Monitoring:
In modern agriculture, data-driven decisions are critical for improving crop production. RL robots can collect vast amounts of data regarding plant health, environmental conditions, and overall farm performance. With this data, farmers can make informed decisions to improve crop management and anticipate potential problems.
The Benefits of Reinforcement Learning in Agriculture
The integration of reinforcement learning in agricultural robotics offers numerous advantages:
Increased Efficiency: Robots that learn from experience can optimize their operations over time, reducing labor costs and improving productivity.
Sustainability: By minimizing the use of harmful pesticides and fertilizers, RL robots contribute to more sustainable farming practices.
Cost Reduction: Automation through RL-driven robotics can help reduce operational costs by replacing manual labor and improving resource management.
Scalability: RL robots can be deployed in large-scale farms, offering a scalable solution for enhancing agriculture productivity globally.
Enhanced Precision: With the ability to learn and adapt, RL robots can perform tasks with high precision, leading to better crop health and higher yields.
Future Outlook
As the technology matures, we can expect RL-powered agricultural robots to become even more sophisticated, capable of performing a wider range of tasks with greater autonomy. With advancements in machine learning algorithms and robotics hardware, the future of agriculture looks promising, with automation leading the way toward a more efficient, sustainable, and profitable farming industry.
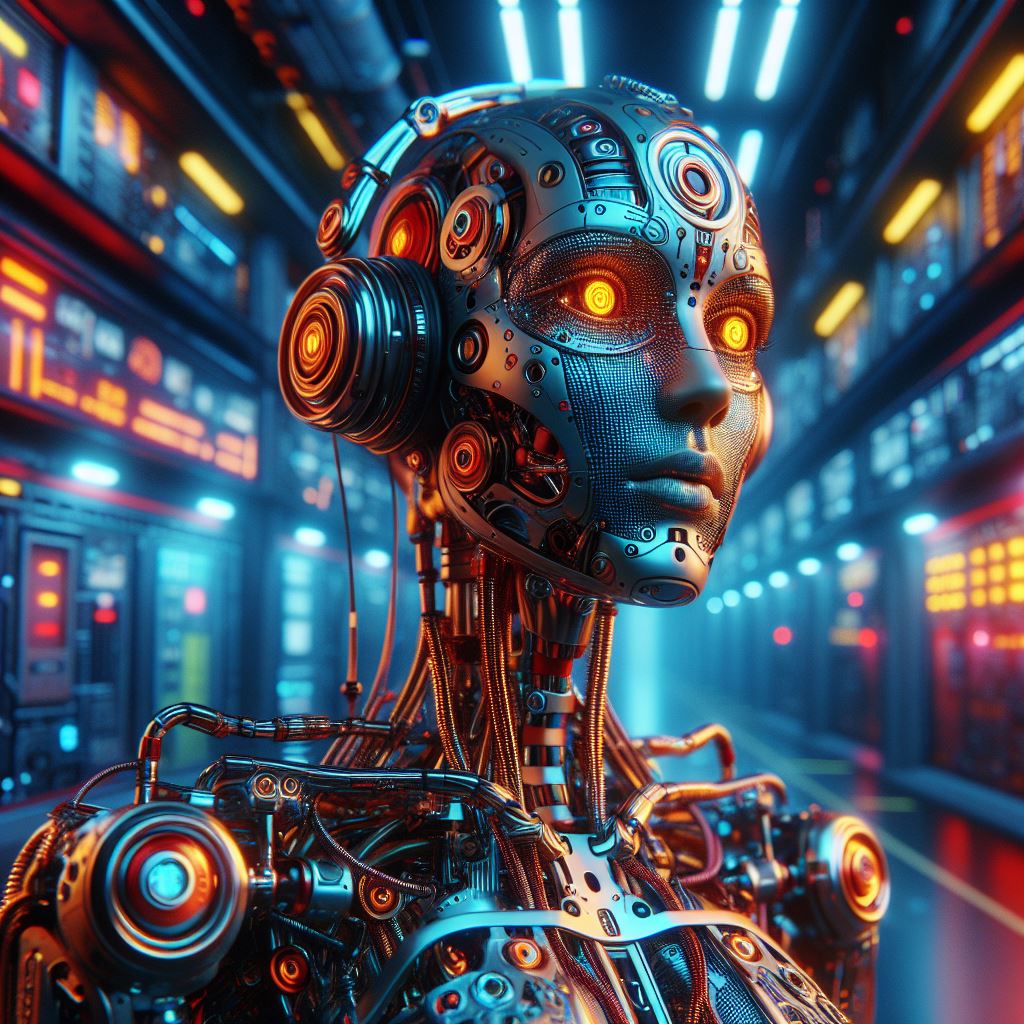