Reinforcement learning (RL) is revolutionizing industries across the globe, and agriculture is no exception. By combining artificial intelligence (AI), robotics, and advanced machine learning techniques, reinforcement learning is paving the way for smarter, more efficient farming practices. This article explores how RL is being used in robotics to optimize agricultural operations, reduce costs, and increase productivity.
What is Reinforcement Learning?
Reinforcement learning is a subset of machine learning where an agent learns to make decisions by interacting with an environment. The agent receives feedback in the form of rewards or penalties based on its actions, which encourages it to take actions that maximize the cumulative reward over time. This learning process mimics the way humans and animals learn through trial and error, making it highly suitable for applications in dynamic, real-world scenarios like agriculture.
Reinforcement Learning in Agricultural Robotics
In the agricultural sector, robots powered by reinforcement learning are beginning to transform how tasks such as planting, harvesting, irrigation, and crop monitoring are performed. These robots use real-time data to continuously learn and adapt to the environment, improving efficiency and accuracy over time.
For instance, RL-based agricultural robots can autonomously navigate through fields, identify the best time to harvest crops, or detect signs of plant diseases. By learning from past experiences and adjusting their behavior accordingly, these robots can optimize farming processes, leading to reduced labor costs and improved crop yields.
Advantages of Reinforcement Learning in Agriculture
Increased Efficiency: With reinforcement learning, robots can make decisions faster and more accurately than humans. This leads to tasks being completed in less time and with fewer errors, enhancing overall operational efficiency.
Cost Reduction: Automation driven by RL reduces the need for human labor and the associated costs. Additionally, these robots can work around the clock, further decreasing operational expenses.
Precision Agriculture: RL robots can perform tasks with high precision, from seeding to fertilization and pest control. This precision ensures better crop health, fewer resources wasted, and optimized yields.
Sustainability: By minimizing the use of pesticides, fertilizers, and water through more targeted actions, RL-based agricultural robots contribute to more sustainable farming practices.
Data-Driven Decision Making: The robots are equipped with sensors and cameras that collect vast amounts of data, which they analyze in real-time. This data-driven approach allows farmers to make more informed decisions about crop management, irrigation, and pest control.
Real-World Applications
Several real-world applications are already benefiting from reinforcement learning in agriculture. For example, companies are developing autonomous tractors and drones that can plant seeds, monitor crop growth, and apply fertilizers and pesticides only where needed. These systems reduce resource usage while maximizing crop health.
One notable example is the use of RL for precision irrigation systems. Reinforcement learning algorithms analyze weather patterns, soil moisture levels, and crop needs to optimize water usage, ensuring crops receive the right amount of water at the right time. This not only conserves water but also helps prevent overwatering, which can lead to crop diseases.
Challenges and Future Outlook
While the potential for RL in agriculture is vast, several challenges remain. Developing robots that can operate autonomously in unpredictable environments is still a complex task. There are also concerns regarding the cost of implementing such advanced technologies, which may be prohibitive for small-scale farmers.
However, as technology continues to advance, these challenges are expected to be overcome. With the potential to drastically improve productivity, reduce environmental impact, and ensure food security, the future of reinforcement learning in agricultural robotics is bright.
Conclusion
Reinforcement learning in robotics is transforming the agricultural industry by introducing smarter, more efficient farming practices. With the ability to reduce costs, increase yields, and promote sustainability, RL-driven robots are poised to become an essential part of the future of agriculture. As technology evolves, these innovations will help meet the growing demands for food in an environmentally responsible manner.
5
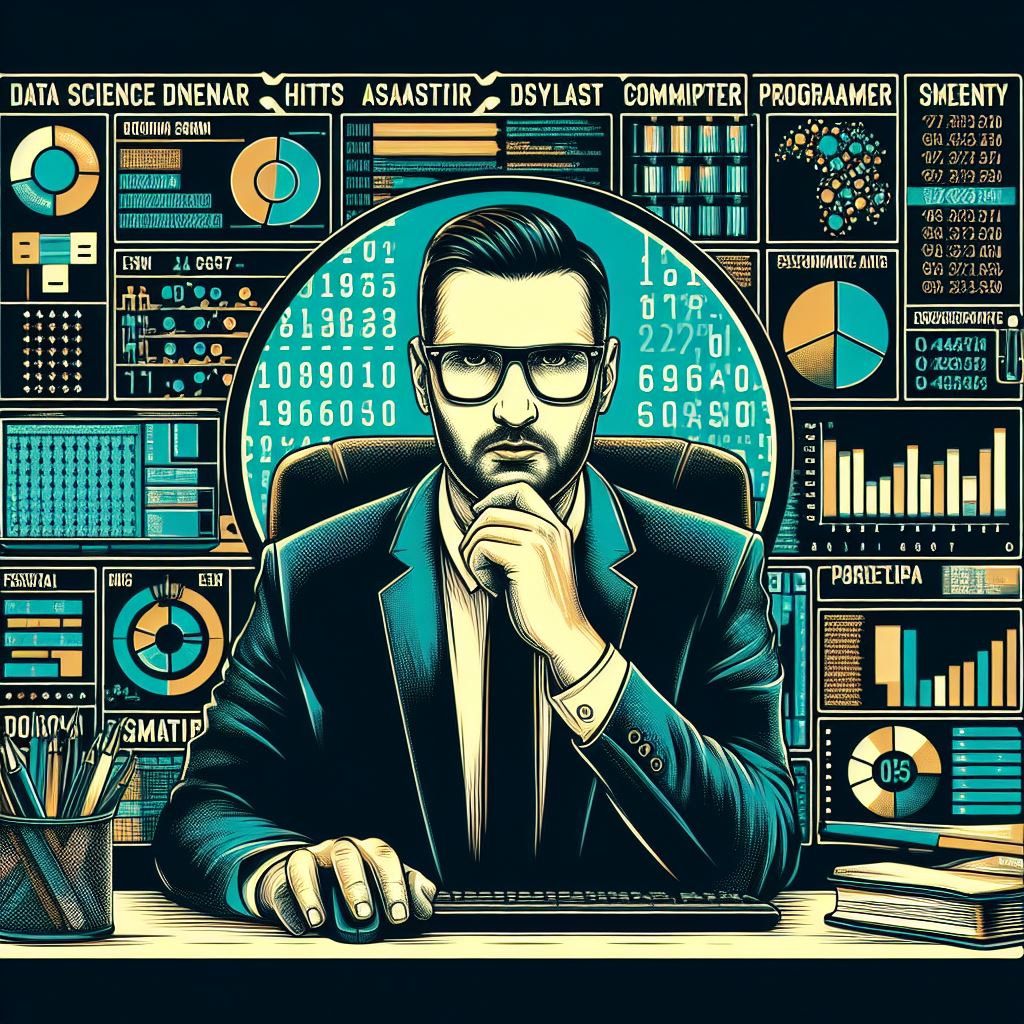