Reinforcement learning (RL), a type of machine learning, is transforming industries by enabling systems to learn from their environment through trial and error. In agriculture, RL combined with robotics is paving the way for more efficient, sustainable farming practices. The fusion of these technologies is not only enhancing productivity but also addressing key challenges in modern farming, such as labor shortages, crop management, and environmental sustainability.
Reinforcement learning is particularly effective in robotics because it empowers robots to make decisions autonomously, optimizing their actions for specific tasks. For instance, an agricultural robot using RL can learn how to navigate a field, avoid obstacles, and identify which areas require attention, all while minimizing energy consumption and resource waste.
The application of RL in agriculture has made significant strides in precision farming, where robots and drones are used to monitor crops, apply fertilizers, and even harvest produce. These robots can adapt their strategies based on the feedback they receive, continually improving their performance and increasing crop yields.
One of the key benefits of RL in agricultural robotics is its ability to enhance the efficiency of crop monitoring. With RL-powered robots, farmers can gather detailed insights into crop health by using sensors to track factors like soil moisture, temperature, and plant growth. This data-driven approach allows farmers to make informed decisions about irrigation, fertilization, and pest control, ultimately improving crop quality and yield while minimizing the use of chemicals.
Another important aspect of RL in agriculture is its potential to address labor shortages in farming. The agricultural industry faces challenges due to a lack of workers, particularly during peak seasons like planting and harvest time. Robotics, powered by RL, can take over time-consuming tasks, such as planting seeds, weeding, and harvesting, thereby reducing the need for manual labor and improving operational efficiency.
In addition to increasing productivity, RL in robotics offers significant environmental benefits. By optimizing farming practices, RL-powered robots can help reduce the carbon footprint of agriculture. They enable more precise application of resources like water, fertilizers, and pesticides, which leads to a reduction in waste and a smaller environmental impact.
Moreover, the ongoing development of RL algorithms and robotic systems continues to push the boundaries of what’s possible in agriculture. From autonomous tractors to drone-powered crop monitoring, the integration of RL and robotics holds great promise for creating smarter, more sustainable farming systems. As technology advances, it is expected that RL-driven robots will become even more adept at navigating complex agricultural tasks, further enhancing efficiency and sustainability.
The future of agriculture lies in the intelligent combination of robotics and machine learning. As these technologies continue to evolve, we can expect to see even greater improvements in farming practices, including increased productivity, reduced environmental impact, and more efficient use of resources.
In conclusion, reinforcement learning is driving innovation in agricultural robotics by enabling robots to make better decisions and optimize farming processes. This transformative technology is helping farmers tackle some of the biggest challenges in agriculture, including labor shortages, resource management, and environmental sustainability. As the agricultural sector embraces RL-powered robotics, the future of farming looks brighter, more efficient, and more sustainable than ever before.
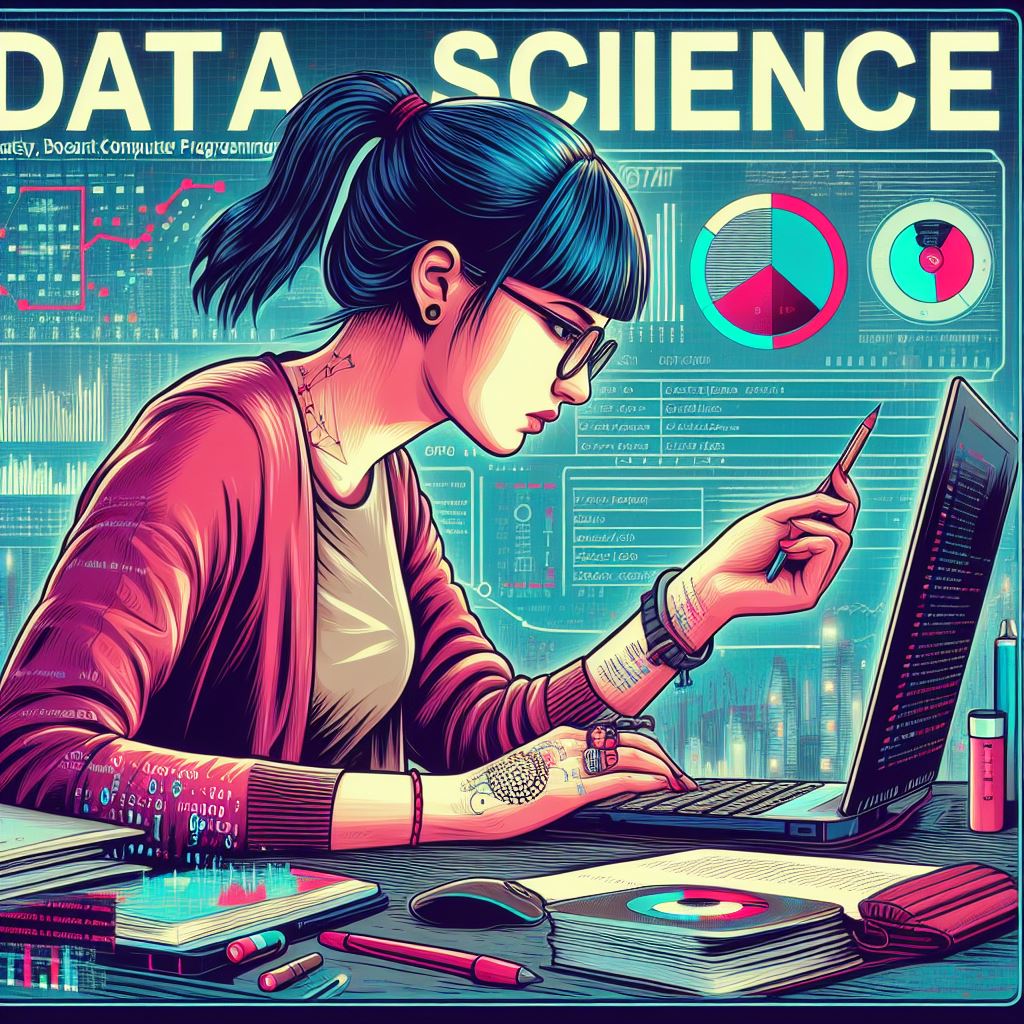