Reinforcement learning (RL) is an area of machine learning that focuses on training algorithms to make a sequence of decisions through trial and error. In recent years, RL has emerged as a game-changer in several industries, with agriculture being one of the most promising sectors benefiting from this technology. The integration of reinforcement learning in robotics is revolutionizing farming practices, enhancing efficiency, sustainability, and productivity in ways previously unimaginable.
What is Reinforcement Learning in Robotics?
Reinforcement learning involves an agent (a robot, for example) learning from its environment by taking actions and receiving feedback in the form of rewards or penalties. Over time, the robot learns to optimize its actions to maximize cumulative rewards. This principle, when applied to robotics, enables machines to autonomously navigate and perform tasks such as harvesting, planting, and monitoring crops.
In agriculture, robots equipped with RL algorithms can be used to perform tasks that traditionally required human labor, offering a solution to the growing shortage of workers in the agricultural sector. These robots can adapt to changing conditions in the field, such as different soil types, weather conditions, or the growth stages of crops, all while minimizing the use of resources like water, fertilizers, and pesticides.
How Reinforcement Learning Benefits Agricultural Robotics
Autonomous Crop Management: One of the key applications of RL in agricultural robotics is autonomous crop management. Robots equipped with RL algorithms can learn to monitor crop health, detect diseases, and identify areas in need of irrigation or fertilization. This allows farmers to reduce the amount of resources used while improving crop yields and quality.
Precision Farming: RL-based robots can be programmed to operate with extreme precision, enabling farmers to apply water, fertilizers, and pesticides only where they are needed. This reduces waste and minimizes environmental impact, contributing to more sustainable farming practices. By learning from each environment, the robots can continuously improve their operations, adapting to different field conditions.
Improved Harvesting Efficiency: Harvesting is one of the most labor-intensive and time-sensitive tasks in agriculture. RL-equipped robots can learn to identify ripe fruits or vegetables and autonomously pick them without damaging the crops. This significantly reduces the need for manual labor and ensures that crops are harvested at the optimal time, preventing spoilage and waste.
Real-Time Decision Making: With reinforcement learning, agricultural robots can make real-time decisions based on dynamic conditions. For instance, if a robot detects that certain crops are not receiving enough sunlight due to shading from nearby plants, it can take corrective action, such as repositioning itself or adjusting the amount of sunlight exposure. This ability to make instant adjustments improves the robot’s effectiveness and efficiency.
Adaptation to Changing Conditions: RL enables robots to continuously adapt to changes in the agricultural environment. Weather patterns, pest infestations, and crop diseases can vary throughout the growing season, and RL-based robots can quickly learn to respond to these changes. This flexibility ensures that robots can operate effectively under a variety of unpredictable conditions.
The Future of RL in Agricultural Robotics
As the demand for food grows and the agricultural sector faces challenges like climate change, labor shortages, and resource scarcity, reinforcement learning in robotics offers a sustainable solution to these problems. The ongoing development of RL algorithms and robotics technologies will likely lead to even more advanced systems capable of performing complex tasks autonomously.
Farmers are already adopting RL-powered robots for tasks like planting, crop monitoring, and harvesting, and as these systems become more efficient and cost-effective, their use will likely expand. In the future, we can expect a greater integration of RL and robotics in agriculture, which will not only boost crop yields but also enhance the overall sustainability and profitability of farming operations.
Conclusion
Reinforcement learning is transforming the agricultural industry by enabling robots to perform a variety of tasks autonomously, efficiently, and sustainably. As technology continues to evolve, RL-powered agricultural robots will play a crucial role in feeding the growing global population while minimizing the environmental impact of farming. The marriage of robotics and reinforcement learning promises to reshape the future of agriculture, offering a smart and sustainable path forward.
5
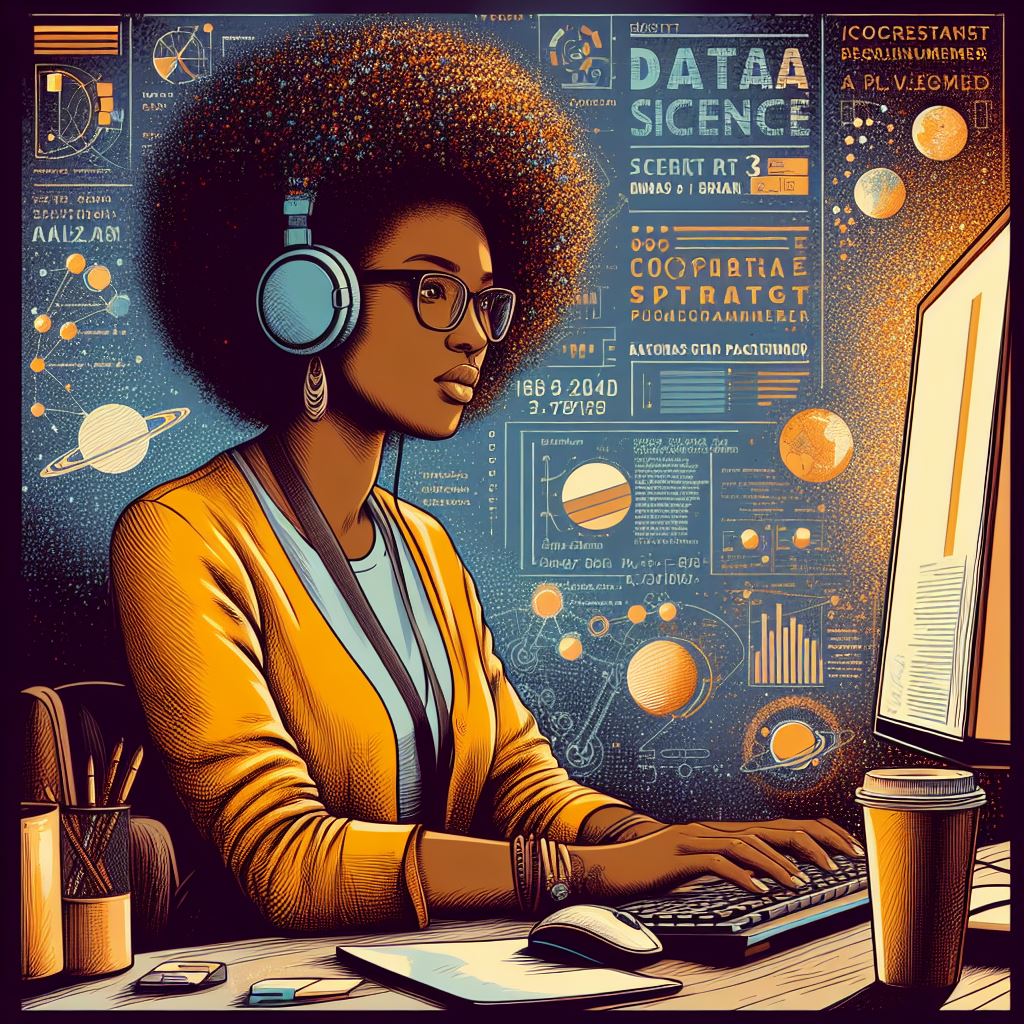