In the ever-evolving field of agriculture, the integration of technology has revolutionized farming practices. One of the most promising advancements is the use of reinforcement learning (RL) in robotics, driving efficiency and precision in agriculture. This cutting-edge technology offers significant potential for automating complex tasks, optimizing resources, and increasing yields while reducing environmental impact.
What is Reinforcement Learning?
Reinforcement learning is a subset of machine learning where an agent learns how to act by performing actions in an environment to achieve a specific goal. The agent is guided by rewards or penalties based on its actions. Over time, the agent learns to make decisions that maximize long-term rewards. In agriculture, RL can be applied to robots that perform tasks like crop monitoring, harvesting, and pest control.
Robotics in Agriculture
Agriculture robots are transforming the way farmers approach labor-intensive tasks. From autonomous tractors to drones, robots can now assist with tasks that were once manual and time-consuming. Robotics enhances efficiency by reducing the need for human labor, minimizing errors, and providing more consistent results. When combined with reinforcement learning, robots become even more intelligent, adapting to various farming conditions and improving their performance over time.
Reinforcement Learning Applications in Agricultural Robotics
Autonomous Harvesting: RL can enable robots to identify the optimal time for harvesting crops. For instance, an RL-based robot can learn to pick fruits with the right level of ripeness, reducing waste and ensuring quality. The robot can also adjust its harvesting strategy based on weather patterns or crop growth stages.
Precision Agriculture: Precision farming focuses on using technology to monitor and manage field variability. By integrating RL with robots, farmers can optimize water usage, reduce pesticide application, and use fertilizers more efficiently. RL models can help robots make decisions in real-time based on data collected from soil sensors, weather reports, and crop health.
Pest Control: RL-powered robots can identify and target pests more effectively than traditional methods. Instead of spraying pesticides across an entire field, which can harm beneficial insects and the environment, RL-driven robots can learn to target specific pests, reducing chemical use and increasing sustainability.
Field Mapping and Monitoring: Robots equipped with RL can autonomously navigate fields, collecting data on soil conditions, crop health, and other environmental factors. Over time, these robots can adjust their strategies to improve data accuracy and make more informed decisions, providing farmers with actionable insights for better crop management.
Weed Management: RL-enabled robots can also assist in weed control by differentiating between crops and weeds. By learning from its environment, a robot can autonomously remove weeds or apply herbicides only to areas where necessary, saving time, reducing costs, and minimizing the environmental impact.
Benefits of Reinforcement Learning in Agricultural Robotics
Efficiency: RL allows robots to learn from their environment and improve their performance, resulting in more efficient farming practices.
Cost Reduction: Automation driven by RL can reduce the need for manual labor and decrease the usage of resources such as water, fertilizers, and pesticides.
Sustainability: By using RL to optimize agricultural processes, farming can become more sustainable, with less waste and better resource management.
Increased Yields: RL-powered robots can make real-time adjustments to their actions, ensuring optimal conditions for crop growth, ultimately leading to higher yields.
Challenges and Future Directions
Despite its potential, the application of RL in agricultural robotics is still in its early stages. There are challenges related to sensor technology, computational power, and the high cost of developing RL-based robots. However, as technology advances and becomes more accessible, the future of RL in agriculture looks promising.
The future may bring even smarter robots capable of performing complex tasks with greater autonomy. Further research and development in this field will continue to push the boundaries of what is possible, ultimately transforming the agricultural industry for the better.
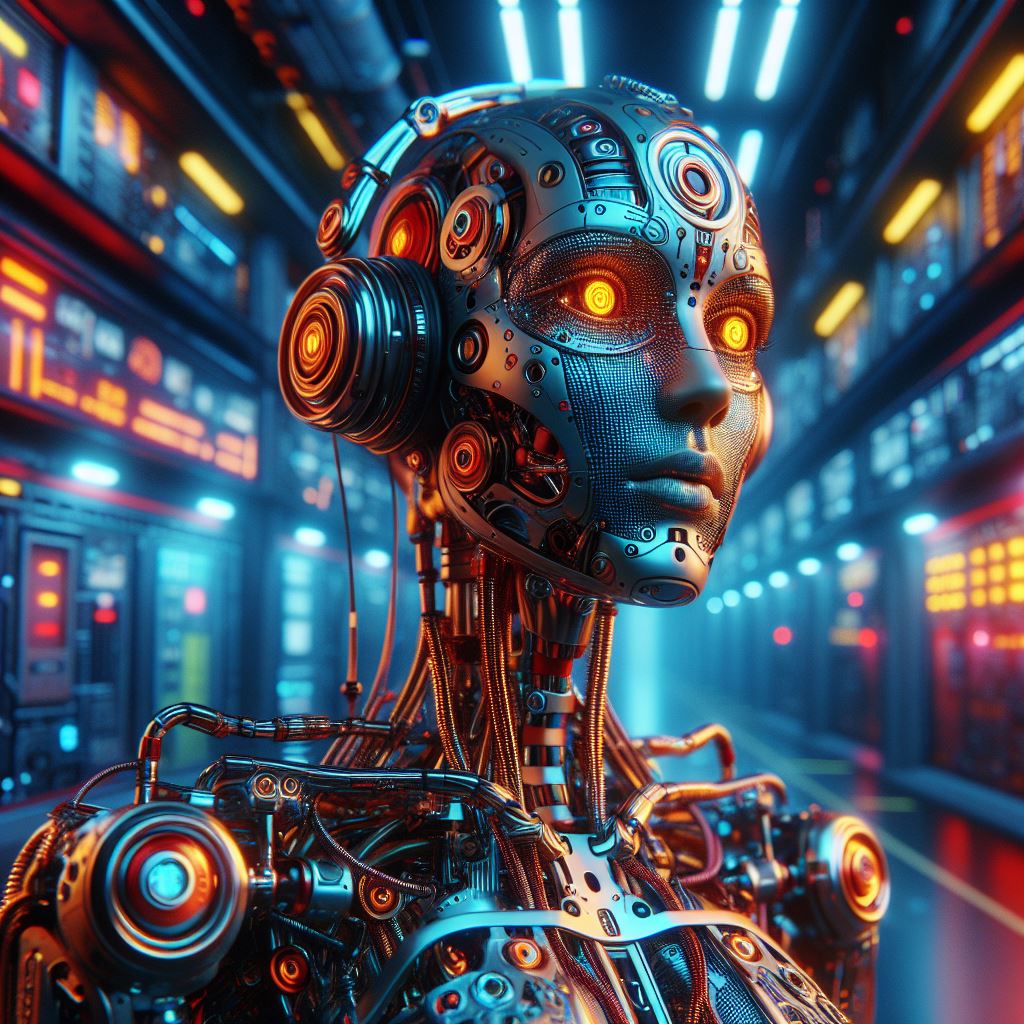