Reinforcement learning (RL) is rapidly transforming various industries, with agriculture being one of the most promising areas. By combining RL with robotics, farmers can automate tasks that were traditionally manual, enhancing productivity and reducing operational costs. This synergy between RL and robotics is paving the way for smarter, more efficient farming practices that address global food security challenges.
Reinforcement learning, a branch of machine learning, works by training algorithms through trial and error, rewarding them for achieving desired outcomes and penalizing for errors. In agriculture, this allows robots and machines to learn optimal strategies for tasks such as planting, harvesting, pest control, and crop monitoring. The ability to make real-time decisions and adapt to environmental conditions is critical, as agricultural environments are often unpredictable and diverse.
One of the key applications of RL in agriculture is autonomous crop harvesting. Using robotic systems, farmers can delegate the task of picking fruits and vegetables to machines that learn from their surroundings. These robots are trained to identify ripe produce, approach them efficiently, and harvest them without damaging the crops. As they interact with the environment, they improve their harvesting techniques through reinforcement learning, becoming more effective with each attempt.
Robotics in agriculture also extends to precision farming, where robots equipped with RL algorithms are deployed to monitor crop health, detect diseases, and optimize resource usage. For instance, drones equipped with computer vision and RL can analyze soil conditions, monitor irrigation systems, and identify nutrient deficiencies. By continuously learning from their environment, these robots can provide tailored solutions that maximize yield while minimizing water usage and fertilizer consumption.
RL-driven robotics are also making strides in weed management. Traditionally, farmers use chemical herbicides to manage weeds, but this method often results in overuse of chemicals and environmental harm. RL-powered robots, on the other hand, can identify and target weeds precisely, applying herbicides only where needed. This not only reduces the use of chemicals but also promotes sustainable farming practices that are more eco-friendly.
The integration of RL and robotics is not without its challenges, however. The agricultural environment is complex, and the development of robots capable of handling such complexity requires substantial investment in research and technology. Training RL algorithms in real-world agricultural settings also demands vast amounts of data and computing power. Nonetheless, as the technology matures, the benefits of RL in agriculture will become increasingly evident.
The future of RL in agriculture looks promising, with ongoing advancements in artificial intelligence, sensor technology, and robotics. As more farmers adopt these innovations, we can expect significant improvements in efficiency, sustainability, and productivity. Reinforcement learning-powered robotics are not just reshaping the way we grow food—they are helping us feed the world in smarter, more sustainable ways.
5
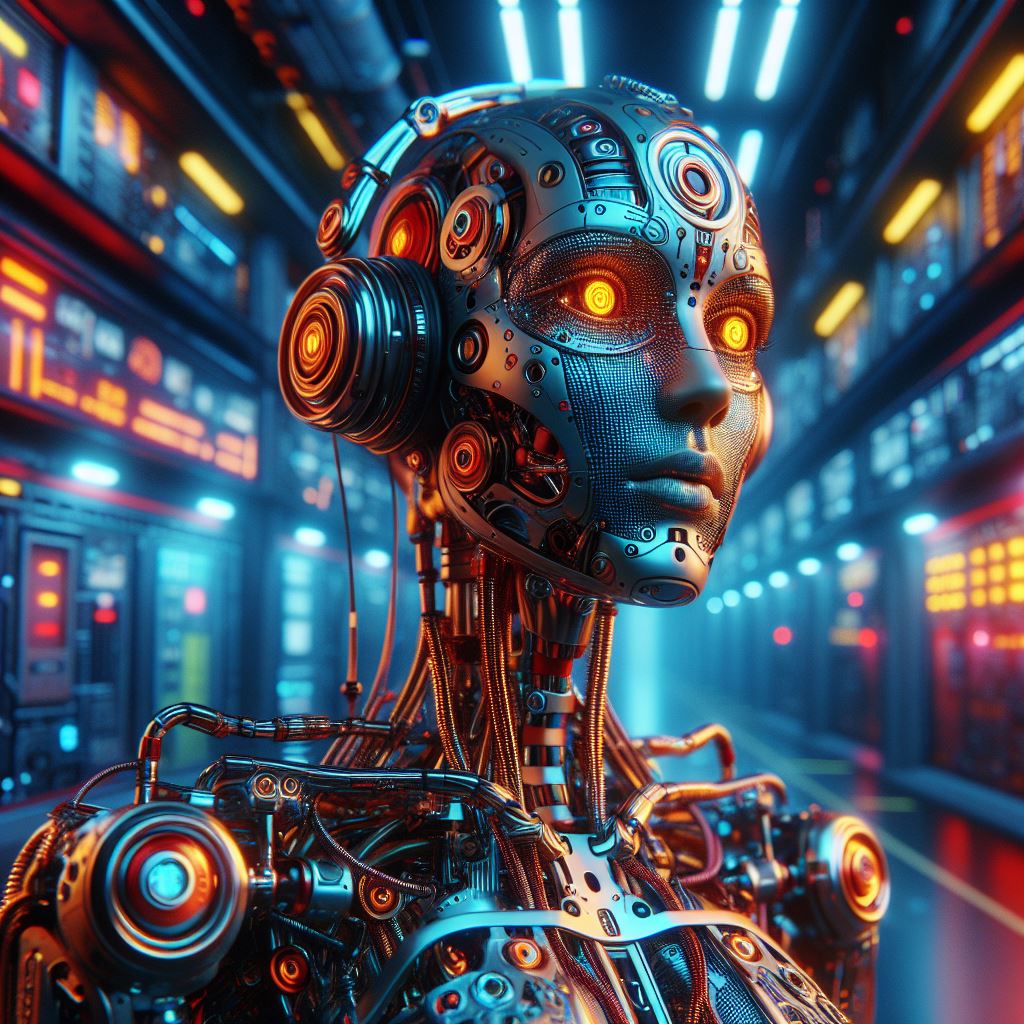