Reinforcement learning (RL) is a machine learning paradigm that allows an agent to learn through trial and error, improving its performance over time. When applied to robotics, RL can empower robots to adapt and make decisions autonomously, making it an invaluable tool in the agricultural sector. This fusion of reinforcement learning and robotics is revolutionizing the way farming operations are managed, increasing efficiency, and reducing human labor.
In modern agriculture, robots are already being used for tasks such as planting, harvesting, weeding, and monitoring crops. However, integrating RL into these robots takes the technology a step further, enabling them to continuously learn from their environment and optimize their behavior. By using RL algorithms, robots can make real-time decisions based on feedback from their surroundings, whether it’s adjusting the path of a tractor or determining the optimal time to harvest crops.
One of the key advantages of RL in agricultural robotics is its ability to handle uncertainty. Farming environments are often unpredictable, with changing weather patterns, soil conditions, and pest infestations. RL enables robots to learn and adapt to these changes, ensuring that they perform optimally even in the face of challenges. For example, an RL-powered drone can adjust its flight pattern in response to wind changes or detect areas of a field that need extra attention due to varying soil conditions.
Robots equipped with RL can also perform tasks that require precision and efficiency, such as planting seeds at the correct depth or spacing them optimally for growth. By using continuous feedback from sensors, the robots refine their actions over time, becoming more accurate and efficient with each iteration. This results in higher yields, better resource utilization, and reduced waste, all of which are essential for sustainable farming practices.
Additionally, RL enables robots to handle tasks that are labor-intensive and time-consuming, such as weeding. Traditional methods of weeding often rely on chemicals, which can have negative environmental impacts. Robots powered by RL, on the other hand, can identify weeds with high precision and remove them mechanically, reducing the need for herbicides and minimizing the environmental footprint of agriculture.
The integration of RL in robotics also paves the way for autonomous agricultural systems. This means that farms can operate with minimal human intervention, significantly reducing labor costs and improving productivity. For instance, autonomous tractors and harvesters can work around the clock, optimizing tasks based on real-time data, and adjusting their actions accordingly to maximize output.
As the technology continues to evolve, the potential applications of RL in agricultural robotics will expand further. With advancements in machine learning algorithms, sensor technology, and computing power, robots will become even more capable of handling a wide range of farming tasks autonomously and efficiently.
In conclusion, the combination of reinforcement learning and robotics holds great promise for the future of agriculture. By leveraging RL, agricultural robots can adapt to changing conditions, optimize their actions, and reduce the environmental impact of farming. This not only leads to increased productivity but also contributes to sustainable practices that will be essential for feeding a growing global population.
5
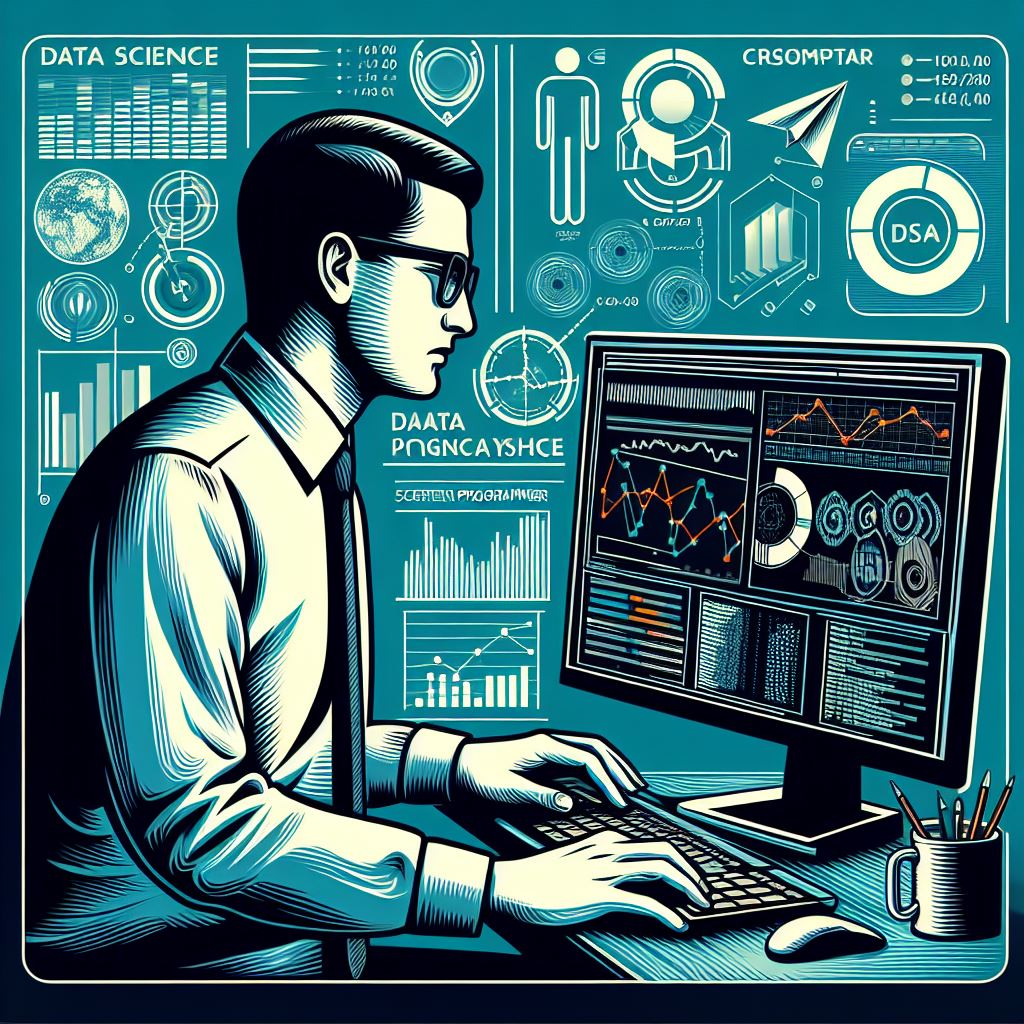