In recent years, the evolution of large language models (LLMs) has brought about transformative advancements in artificial intelligence, enabling businesses to automate complex tasks, improve decision-making, and enhance customer experiences. One of the most exciting innovations in this field is the concept of Retrieval-Augmented Generation (RAG), which combines the power of LLMs with external information retrieval to deliver more accurate, contextually relevant, and insightful responses.
What is Retrieval-Augmented Generation (RAG)?
Retrieval-Augmented Generation (RAG) is an AI technique that combines traditional generative models, like GPT, with external databases or documents to improve the quality and relevance of generated content. While LLMs are powerful at generating text based on learned patterns, they can sometimes lack the ability to access up-to-date or domain-specific information that could enhance their responses. RAG overcomes this by retrieving relevant documents or data points from external sources before generating an answer, ensuring that the output is both precise and relevant to the given context.
How Does RAG Improve LLMs?
LLMs are typically trained on vast amounts of text data, but they have limitations, such as an inability to access real-time information or domain-specific knowledge that may not be represented in the training data. RAG enhances LLMs by incorporating an external knowledge base, such as documents, databases, or search engines, to inform the model’s response.
When a user poses a question or provides a prompt, the RAG system first retrieves relevant pieces of information from its external source. These documents are then fed into the LLM, which uses them as additional context to generate a more informed and accurate answer. This process allows the model to provide contextually rich, fact-based, and highly relevant responses, rather than relying solely on the information stored during its initial training.
The Benefits of RAG for Business Solutions
Improved Accuracy: By retrieving relevant external documents, RAG allows LLMs to generate more accurate and up-to-date information, especially when it comes to specialized topics or fast-changing industries like finance, healthcare, and technology.
Contextual Relevance: RAG helps ensure that the answers generated are context-specific, improving the model’s understanding of a particular domain or query. This is especially beneficial in business scenarios where detailed, precise information is crucial.
Enhanced User Experience: With RAG, businesses can offer more intelligent AI-powered solutions that can answer complex questions, summarize lengthy documents, and offer insights into niche topics. This leads to a better user experience and can save time in industries like customer support, legal, and research.
Scalability in Business Applications: As businesses scale, the ability to continuously update their knowledge base with RAG becomes essential. Whether it’s incorporating customer feedback, the latest market trends, or new research, businesses can ensure their AI systems are always using the most relevant and up-to-date information.
Cost Efficiency: By reducing the need for human intervention in answering queries or analyzing documents, RAG-powered LLMs can automate tasks that were previously time-consuming and resource-intensive. This leads to cost savings and allows businesses to focus their resources on other strategic goals.
Real-World Applications of RAG and LLMs
In the business world, RAG-enhanced LLMs are already being used across various industries. For instance, in legal tech, RAG can help generate accurate legal documents by retrieving relevant case law or statutes before generating text. In customer service, businesses can use RAG to pull data from previous interactions, customer queries, or product manuals to deliver faster and more personalized responses. Similarly, in finance, RAG can assist in analyzing market trends and producing reports based on the latest data.
Conclusion
The combination of Retrieval-Augmented Generation (RAG) and large language models (LLMs) presents a powerful opportunity for businesses to leverage AI in a more dynamic and responsive way. By providing more accurate, contextually aware, and real-time data-driven insights, RAG significantly enhances the capabilities of LLMs, making them an indispensable tool for a variety of business applications. As AI continues to evolve, the integration of RAG into business workflows is likely to become increasingly common, enabling companies to stay ahead in an ever-competitive landscape.
5
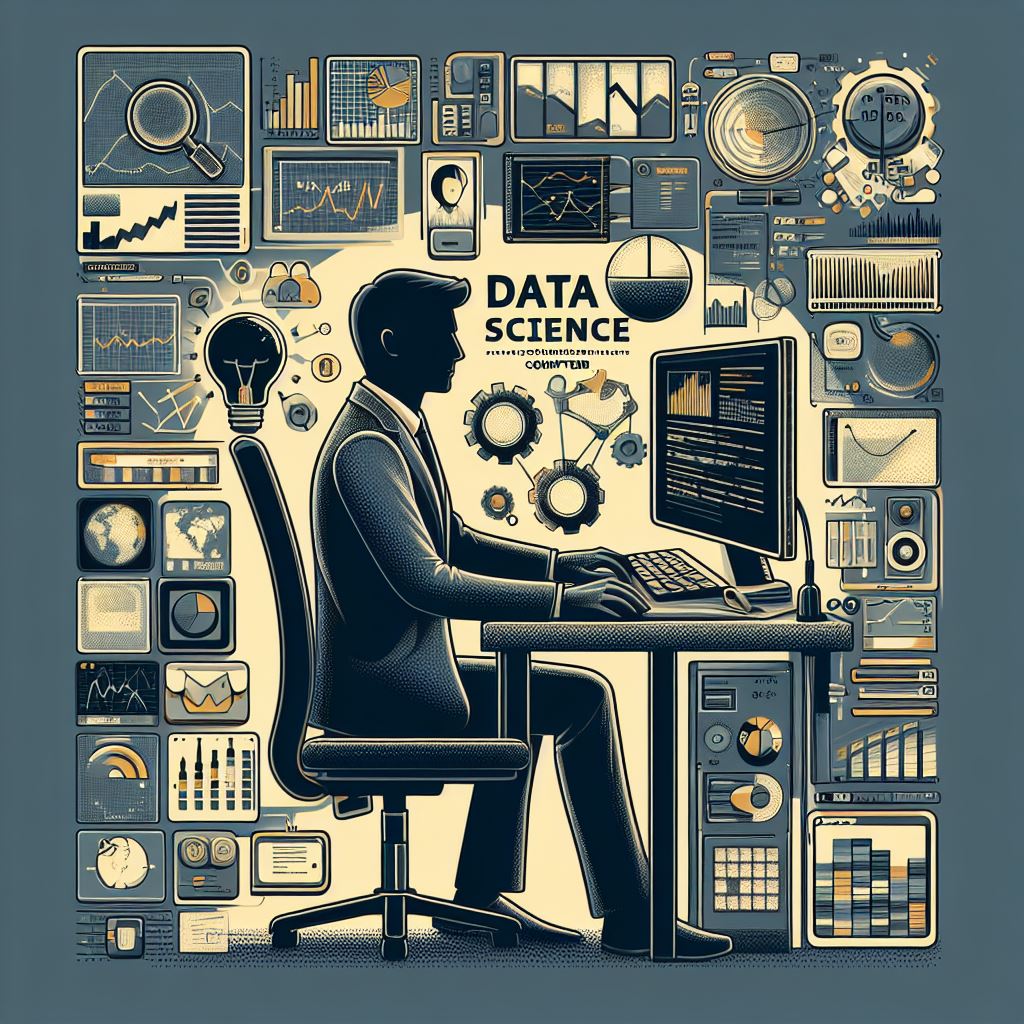