The intersection of reinforcement learning (RL), robotics, and agriculture is revolutionizing the way we approach farming. As the agricultural industry faces numerous challenges—ranging from labor shortages to increasing food demand—innovative solutions are necessary to sustain growth. Reinforcement learning, a branch of machine learning where agents learn to make decisions by interacting with an environment, is emerging as a game-changer in agricultural robotics.
Reinforcement learning allows robots to autonomously make decisions based on real-time data. This ability is particularly useful in farming, where conditions can change rapidly, and traditional methods may be slow to adapt. By integrating RL into agricultural robots, these machines can optimize tasks such as crop monitoring, irrigation, pest control, and harvesting. In this article, we’ll explore how reinforcement learning is shaping the future of robotics in agriculture.
1. Autonomous Crop Monitoring
Traditional crop monitoring methods, such as manual inspections or aerial surveys, can be labor-intensive and often fail to provide real-time insights. With RL-powered robots, farmers can achieve continuous and efficient monitoring. These robots are equipped with sensors and cameras that collect data on crop health, soil moisture, and pest activity. Through RL algorithms, these robots learn to analyze the data and make decisions on where to focus their efforts.
For example, an RL-based robot might identify an area with insufficient water supply and decide to deliver precise irrigation, improving water conservation. The ability to monitor crops autonomously ensures better yields and reduces the need for manual labor.
2. Optimizing Irrigation Systems
Irrigation is a critical aspect of agriculture, especially in regions with water scarcity. Using RL, robots can optimize irrigation schedules based on real-time environmental conditions, such as temperature, humidity, and soil moisture. Through continual learning, these robots improve their decision-making abilities over time, ensuring that water is applied efficiently and reducing waste.
An RL-powered irrigation system can adapt to weather patterns and soil changes, ensuring crops receive just the right amount of water. This not only conserves resources but also enhances crop health and reduces operational costs.
3. Precision Pest Control
Pest infestations can cause significant damage to crops, and traditional pest control methods often involve blanket pesticide applications. This approach is not only inefficient but also harmful to the environment. Robotics, combined with RL, offers a more targeted approach to pest control.
Robots powered by RL can autonomously identify and target pests, applying treatments only when and where necessary. These robots are equipped with computer vision and machine learning algorithms to detect pest behavior and locations. Over time, the RL system learns the most effective methods for pest eradication, reducing pesticide use and minimizing environmental impact.
4. Efficient Harvesting
Harvesting is one of the most labor-intensive tasks in agriculture. Traditionally, it relies on human workers or large machinery, both of which can be expensive and inefficient. RL-based robots are revolutionizing this process by offering more efficient and cost-effective solutions.
RL-powered harvesters learn how to identify ripe crops and determine the best harvesting techniques. These robots can work autonomously or in coordination with other machines to optimize the harvest process, reducing waste and improving crop yields. Additionally, the use of robots in harvesting can help mitigate labor shortages, ensuring that crops are harvested at their peak.
5. Future Potential of RL in Agriculture
The integration of reinforcement learning in agriculture is still in its early stages, but the potential for growth is immense. As the technology continues to improve, we can expect more advanced RL-driven robots that are capable of performing a wider range of tasks. From planting and fertilizing to climate adaptation and sustainability, RL-powered robots will play a critical role in the future of farming.
By increasing efficiency, reducing waste, and improving decision-making, reinforcement learning is helping farmers address some of the most pressing challenges in agriculture. The combination of robotics and RL is not just enhancing productivity but also driving the future of sustainable farming.
5
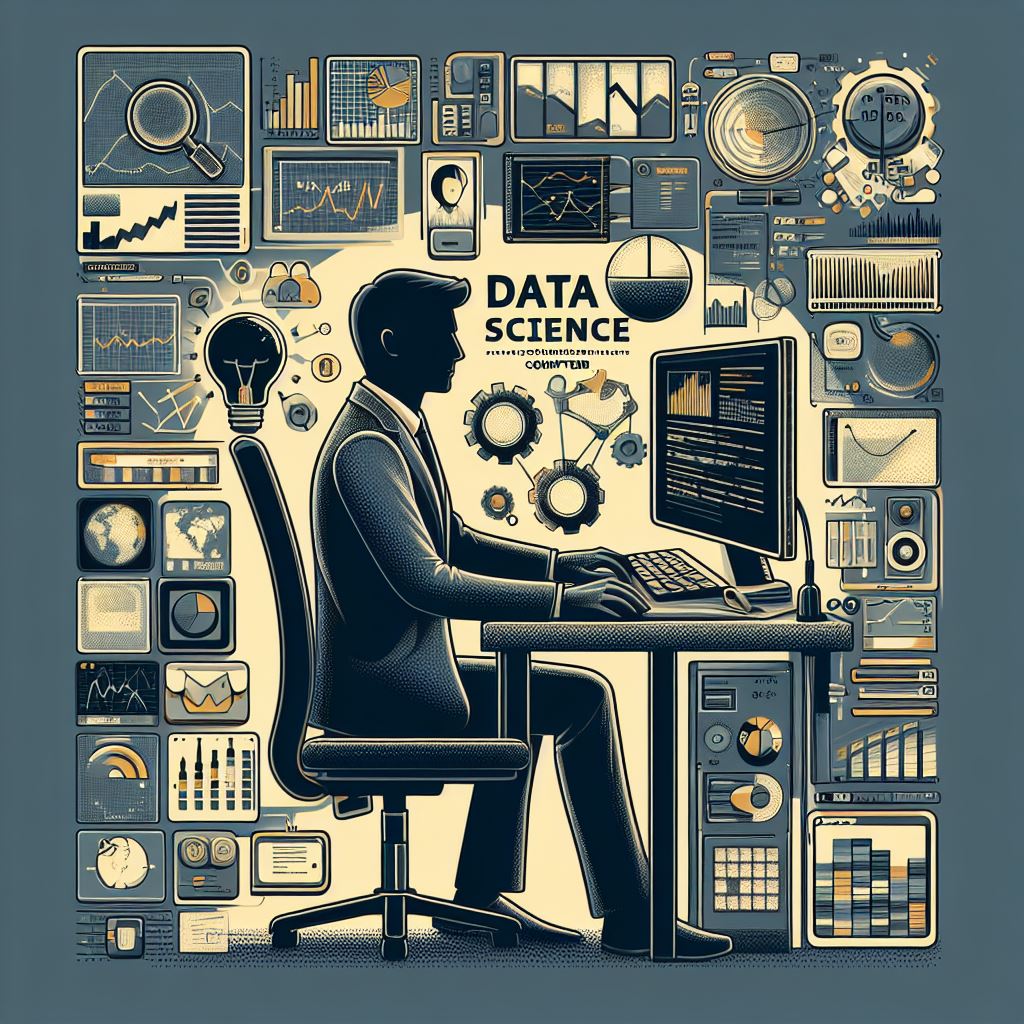