In the rapidly advancing field of artificial intelligence (AI), two technologies stand out for their transformative potential: Retrieval-Augmented Generation (RAG) and Large Language Models (LLM). These innovations are reshaping how AI systems process and generate information, significantly improving their performance in question-answering tasks. Understanding the roles of RAG and LLM is crucial for businesses and developers looking to harness the power of AI in real-world applications.
What is Retrieval-Augmented Generation (RAG)?
RAG is an AI technique that combines retrieval-based methods with generative models, such as large language models (LLMs). The process involves two main components: a retriever and a generator. First, the retriever searches through a large corpus of text to find relevant documents or passages that can answer a given question. Then, the generative model (typically an LLM) is used to generate a coherent and contextually accurate response based on the retrieved information.
The primary advantage of RAG is its ability to provide accurate and context-specific answers. Traditional LLMs can sometimes generate plausible but incorrect answers due to their reliance on pre-trained knowledge. However, by integrating real-time retrieval from relevant sources, RAG enhances the reliability of the generated answers, ensuring they are both relevant and factually correct.
What is a Large Language Model (LLM)?
A Large Language Model (LLM) refers to a deep learning model trained on vast amounts of text data. LLMs, like GPT (Generative Pre-trained Transformer), are designed to understand and generate human-like text based on the context provided by the user. These models are capable of completing sentences, answering questions, translating languages, and even writing essays.
LLMs are pre-trained on diverse datasets, giving them a broad understanding of language and knowledge. However, they often struggle with domain-specific queries or information not included in their training data. This is where RAG comes into play by augmenting the LLM with real-time retrieval capabilities, ensuring that the model can access up-to-date and relevant data.
The Synergy of RAG and LLM in AI Question-Answering
When combined, RAG and LLM create a powerful question-answering system that offers a significant improvement over traditional AI models. RAG ensures that the AI can retrieve the most relevant data from a large corpus before generating a response, while LLMs bring sophisticated language processing and generation capabilities to the table.
This synergy allows AI systems to answer questions more accurately, providing highly relevant responses backed by specific sources. For example, in a business context, a question about market trends could be answered with up-to-date data from industry reports, academic papers, or news articles, retrieved in real-time by the AI and synthesized into a coherent response by the LLM.
Applications of RAG and LLM in Business
The combination of RAG and LLM holds immense potential for businesses across various industries. In customer support, for instance, AI-driven chatbots powered by RAG and LLM can provide detailed and contextually accurate responses to customer inquiries. This helps reduce human intervention and improves customer satisfaction.
Additionally, in sectors like healthcare, finance, and law, where precision and up-to-date information are crucial, RAG and LLM can help professionals access relevant research, guidelines, and regulations in real-time, enhancing decision-making processes.
The Future of RAG and LLM
As AI continues to evolve, the integration of RAG and LLM will likely become more sophisticated. With advancements in retrieval algorithms and generative models, future systems will be able to provide even more precise, context-aware answers, improving the accuracy of AI-driven solutions.
Businesses and developers should begin exploring how these technologies can enhance their AI applications, making the most of the synergy between retrieval and generation. As RAG and LLM continue to develop, they will be integral to the future of AI-driven question-answering systems.
5
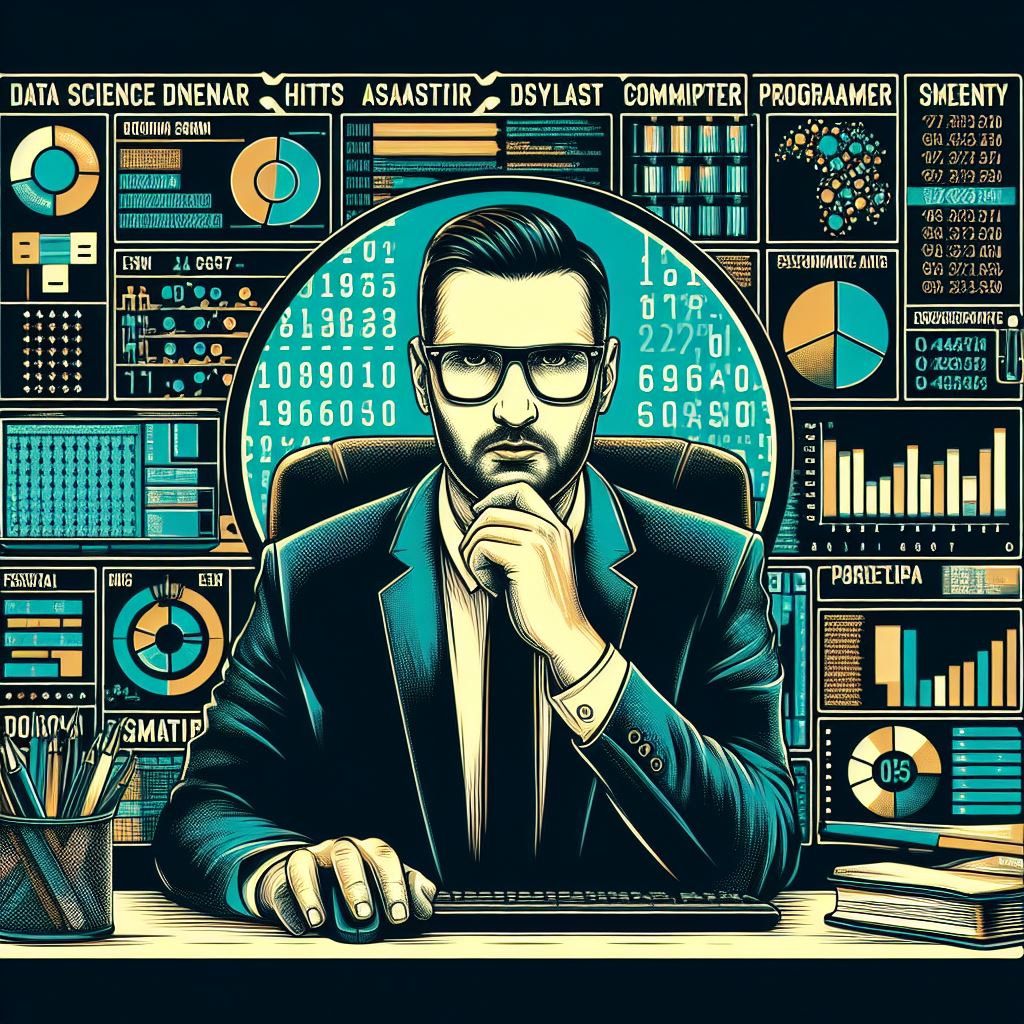