In the age of digital transformation, machine learning (ML) has made its way into various industries, and financial analytics is no exception. Today, individuals can leverage machine learning models for more accurate predictions, better risk management, and more efficient decision-making in their financial activities. By incorporating ML into personal finance strategies, individuals are able to predict market trends, optimize investments, and forecast financial outcomes with unprecedented precision.
What is Machine Learning in Financial Analytics?
Machine learning refers to a subset of artificial intelligence where algorithms are trained on data to identify patterns and make decisions without human intervention. In financial analytics, ML models are applied to large datasets, such as market trends, historical performance data, and financial statements, to generate insights and forecasts that are otherwise difficult for the human mind to process.
How Machine Learning Transforms Financial Forecasting for Individuals
Financial forecasting has traditionally been done using statistical models and historical data. While these methods can offer valuable insights, they are often limited in their ability to capture the complexity and unpredictability of financial markets. Machine learning, on the other hand, enables the development of dynamic models that can adapt to changing conditions and learn from new data over time.
One key area where ML is making an impact is in stock market prediction. Traditional stock market forecasting often relies on technical indicators or simple statistical analysis. However, ML models, such as neural networks or decision trees, can identify complex patterns in the data, offering more reliable and accurate predictions.
For individuals, this means better decisions regarding investments, savings, and expenditures. ML models can forecast trends in stock prices, commodity values, and even real estate prices, enabling investors to make informed choices based on the predictions.
Benefits of Machine Learning in Personal Finance
Enhanced Investment Strategies: ML algorithms can analyze market trends and past performance to offer tailored investment advice. Individuals can use these models to create diversified portfolios that maximize returns while minimizing risks. Furthermore, ML models can suggest optimal investment times based on historical data and current trends.
Accurate Risk Management: One of the most significant challenges for individuals managing their finances is understanding and mitigating risks. ML models can analyze a broad range of financial data to assess the risk associated with specific investments, offering insights into how to better allocate assets for risk-averse individuals.
Personalized Financial Planning: With the ability to process vast amounts of data, machine learning can help individuals create personalized financial plans based on their income, expenses, goals, and risk tolerance. By continuously learning from new data, these models can adjust plans in real time to reflect changing circumstances.
Predicting Market Movements: By training on historical data, ML models can predict market trends and fluctuations, enabling individuals to adjust their strategies proactively. Whether it’s anticipating a downturn in the stock market or predicting interest rate hikes, these predictions give individuals a competitive edge in managing their finances.
Fraud Detection and Prevention: Machine learning plays a crucial role in detecting fraudulent financial activities. By analyzing patterns in transactions, ML models can flag suspicious behavior, helping individuals protect themselves from financial scams and identity theft.
How to Use Machine Learning for Personal Financial Forecasting
Individuals interested in leveraging ML for their personal finances can use a variety of tools and platforms designed for non-experts. Many robo-advisors and financial planning apps now incorporate ML algorithms to provide personalized financial advice and forecasting.
For those with more technical skills, Python libraries such as Scikit-learn, TensorFlow, and Keras offer the ability to build and train custom ML models for financial analysis. There are also pre-built machine learning models available for individuals to experiment with, simplifying the process of integrating ML into financial forecasting.
Conclusion
Machine learning is changing the way individuals approach financial forecasting and analysis. With its ability to process vast amounts of data and identify patterns, ML offers individuals the tools they need to make more informed decisions, optimize investments, and reduce financial risks. By adopting machine learning in their personal finance strategies, individuals can gain a competitive advantage in managing their wealth and securing their financial future.
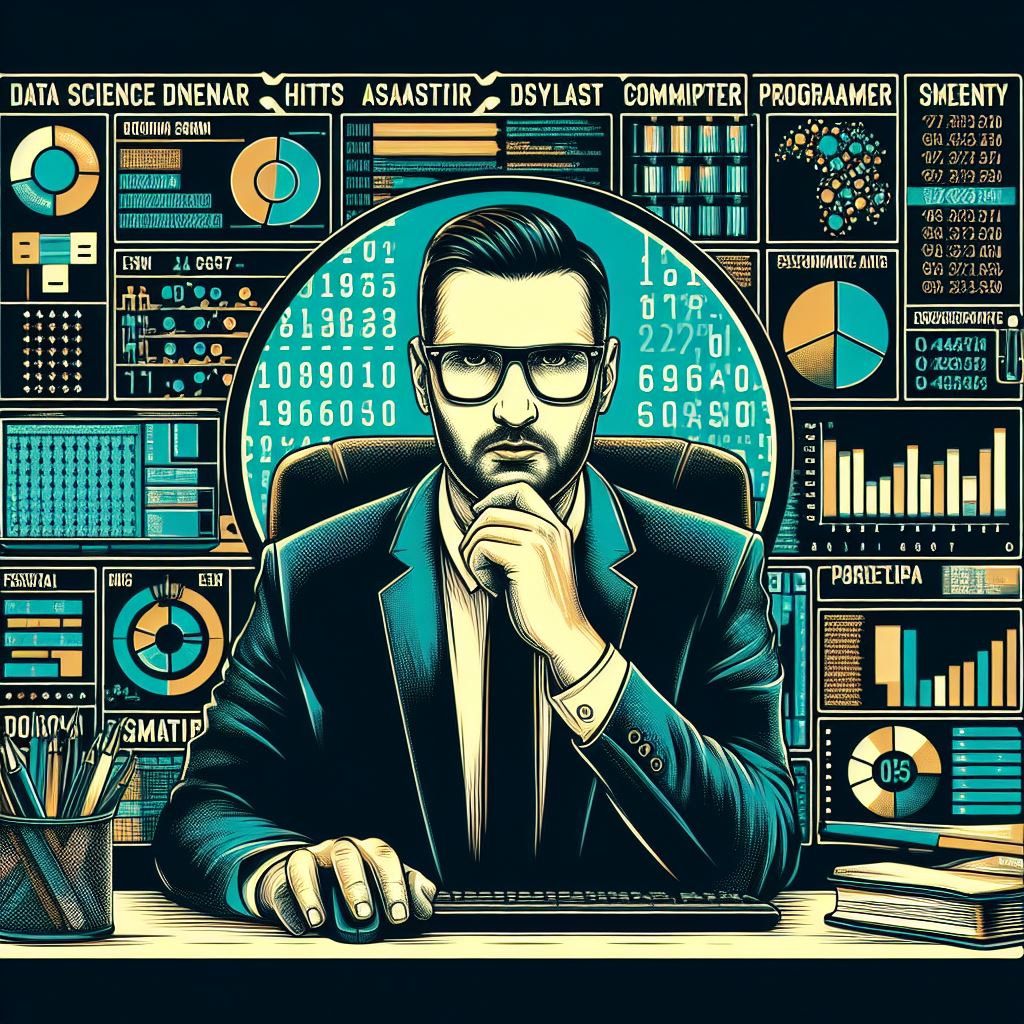