Machine learning (ML) is transforming industries across the globe, and one area where its impact is particularly profound is in financial analytics and forecasting. Traditionally, individuals have relied on financial advisors and static tools to make investment decisions or assess their financial health. However, with the advent of ML, individuals now have access to powerful, data-driven tools that can optimize their financial strategies and predict market trends with remarkable accuracy.
Financial analytics involves the use of statistical methods and tools to analyze financial data, identify trends, and make decisions based on that information. Machine learning, however, takes this a step further by allowing algorithms to automatically learn from data patterns, improving accuracy and efficiency over time. By incorporating ML into financial analytics, individuals can gain insights that were previously available only to large corporations and institutional investors.
One of the most popular applications of ML in financial forecasting is predicting stock market trends. ML algorithms can process vast amounts of historical stock data, identify patterns, and generate forecasts on stock performance. These algorithms can even adapt to new data, ensuring that the forecasts remain relevant and accurate. This means that individuals, even without a financial background, can use ML-powered tools to make informed investment decisions.
Moreover, ML can also help individuals with personal finance management. By analyzing spending patterns and categorizing transactions, ML algorithms can offer personalized recommendations on budgeting and saving. This is particularly useful for people looking to optimize their financial planning and make smarter decisions regarding debt repayment or savings goals.
Another exciting application of ML is in credit scoring and loan approvals. Traditionally, credit scores have been based on a set of rigid criteria, such as credit history and income. However, ML models can take a more holistic approach by analyzing a broader range of factors, such as spending habits and social behavior. This enables more accurate assessments of an individual’s creditworthiness, which can be especially beneficial for those with limited or unconventional credit histories.
Risk management is another area where ML has made significant strides. ML models can assess an individual’s investment portfolio, analyze market conditions, and identify potential risks. By predicting future price movements and market fluctuations, ML tools can help individuals diversify their investments, mitigate risks, and optimize their portfolios for long-term growth.
For individuals looking to explore machine learning in financial forecasting, there are several tools and platforms available. Many online platforms offer user-friendly ML models that require little to no technical expertise. These platforms allow individuals to input their financial data, and the ML models will process the information and provide actionable insights, forecasts, and recommendations. Some tools even allow for the integration of personal financial data, such as bank statements and transaction histories, for more tailored analysis.
Despite the vast potential of ML in financial analytics, it is important for individuals to understand that no system is infallible. While ML models can improve predictions and help optimize decision-making, they are not guaranteed to be 100% accurate. Therefore, it is essential to use these tools in conjunction with traditional financial wisdom and professional advice.
In conclusion, machine learning is reshaping the way individuals approach financial analytics and forecasting. By providing powerful, data-driven insights, ML enables smarter, more informed financial decisions. Whether it’s predicting stock prices, managing personal finances, assessing creditworthiness, or managing risk, ML offers unparalleled opportunities for individuals to enhance their financial strategies. As these technologies continue to evolve, individuals will increasingly have access to advanced tools that put them on par with institutional investors.
5
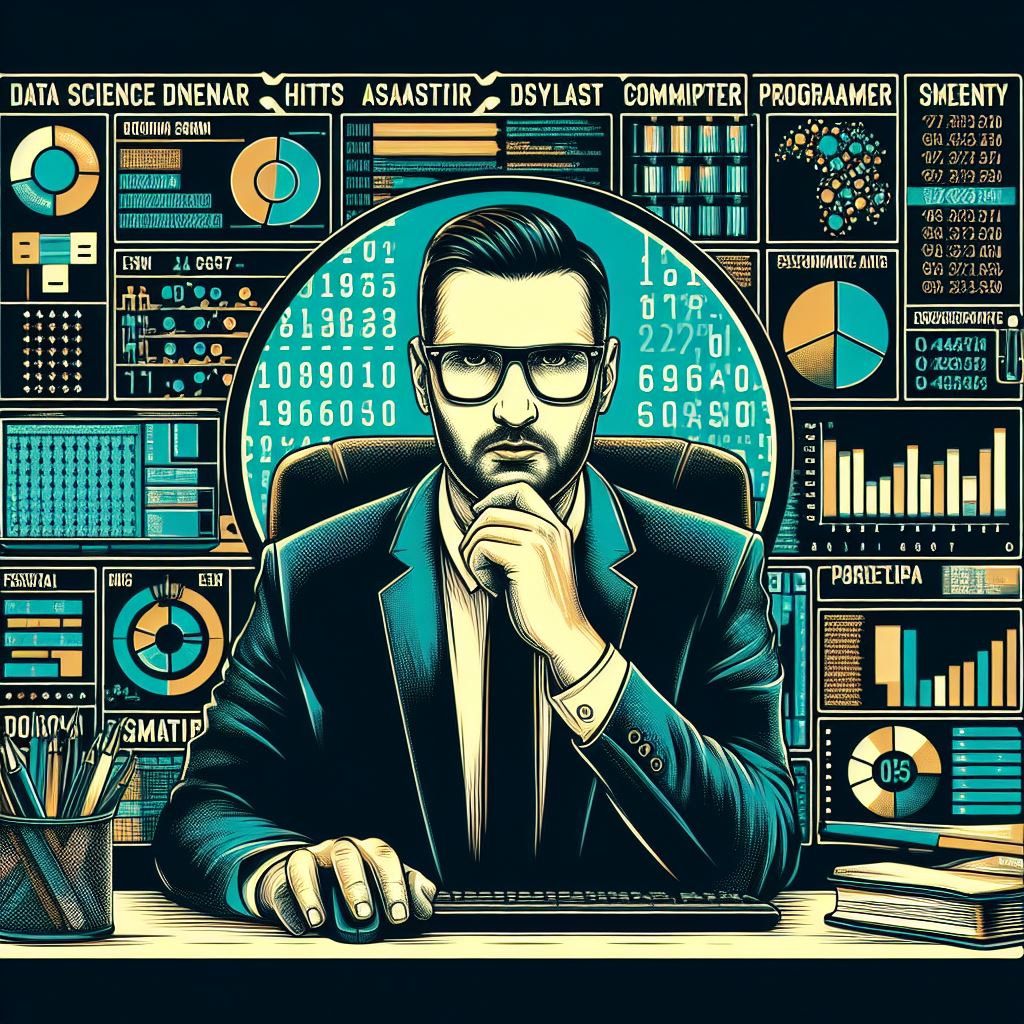