Machine Learning (ML) is transforming various industries, and healthcare is no exception. By leveraging ML, healthcare providers can enhance patient care, optimize medical practices, and streamline operations. With its ability to analyze large volumes of data and recognize patterns, ML has become a vital tool in diagnosing diseases, predicting health outcomes, and improving overall healthcare management.
1. Disease Diagnosis and Detection One of the most prominent ML applications in healthcare is in disease diagnosis. Traditional diagnostic methods often require extensive time and human expertise. ML algorithms, however, can quickly process medical images, patient records, and lab results to assist in diagnosing conditions such as cancer, heart disease, and neurological disorders. For instance, deep learning models can analyze medical imaging, like X-rays and MRIs, with remarkable accuracy, often detecting abnormalities that may be missed by the human eye. This early detection can lead to timely interventions, improving patient outcomes.
2. Predictive Analytics for Patient Outcomes ML algorithms are also being used to predict patient outcomes by analyzing historical medical data. These predictive models help healthcare providers identify individuals at high risk for certain diseases or conditions, such as diabetes, stroke, or heart failure. By assessing a patient’s medical history, lifestyle factors, and genetic predispositions, ML can generate risk scores that guide preventive care strategies. Early intervention based on these predictions can significantly reduce healthcare costs and improve patient quality of life.
3. Personalized Treatment Plans Another exciting application of ML in healthcare is in the development of personalized treatment plans. By analyzing data from various sources, such as electronic health records (EHRs), genetic testing, and lifestyle factors, ML algorithms can tailor treatments to individual patients. This personalized approach not only increases the effectiveness of medical interventions but also minimizes the risk of adverse drug reactions. For example, ML models are being used to recommend personalized drug regimens for cancer patients based on their unique genetic makeup, improving treatment outcomes.
4. Medical Research and Drug Discovery ML is also revolutionizing the field of medical research and drug discovery. Traditionally, drug development is a time-consuming and costly process, with a high rate of failure. ML can accelerate this process by analyzing vast amounts of biological data, such as genomic sequences and chemical structures, to identify potential drug candidates. Additionally, ML models can predict the effectiveness of drugs on various patient populations, enabling more efficient clinical trials. By streamlining the research and development process, ML is helping bring new therapies to market faster and more cost-effectively.
5. Healthcare Operations and Efficiency Beyond patient care, ML is improving healthcare operations and efficiency. Hospital management systems are utilizing ML algorithms to optimize scheduling, resource allocation, and inventory management. Predictive models can forecast patient admissions, allowing hospitals to allocate resources more efficiently and reduce wait times. Additionally, ML is being used to improve supply chain management by predicting demand for medical supplies, ensuring that healthcare facilities are adequately stocked without overstocking. This reduces waste and lowers costs while maintaining quality care.
Conclusion Machine learning applications in healthcare are paving the way for more efficient, accurate, and personalized medical care. From enhancing disease diagnosis to optimizing hospital operations, the potential for ML in healthcare is vast. As technology continues to evolve, we can expect even more groundbreaking applications that will not only improve patient outcomes but also revolutionize the way healthcare is delivered worldwide.
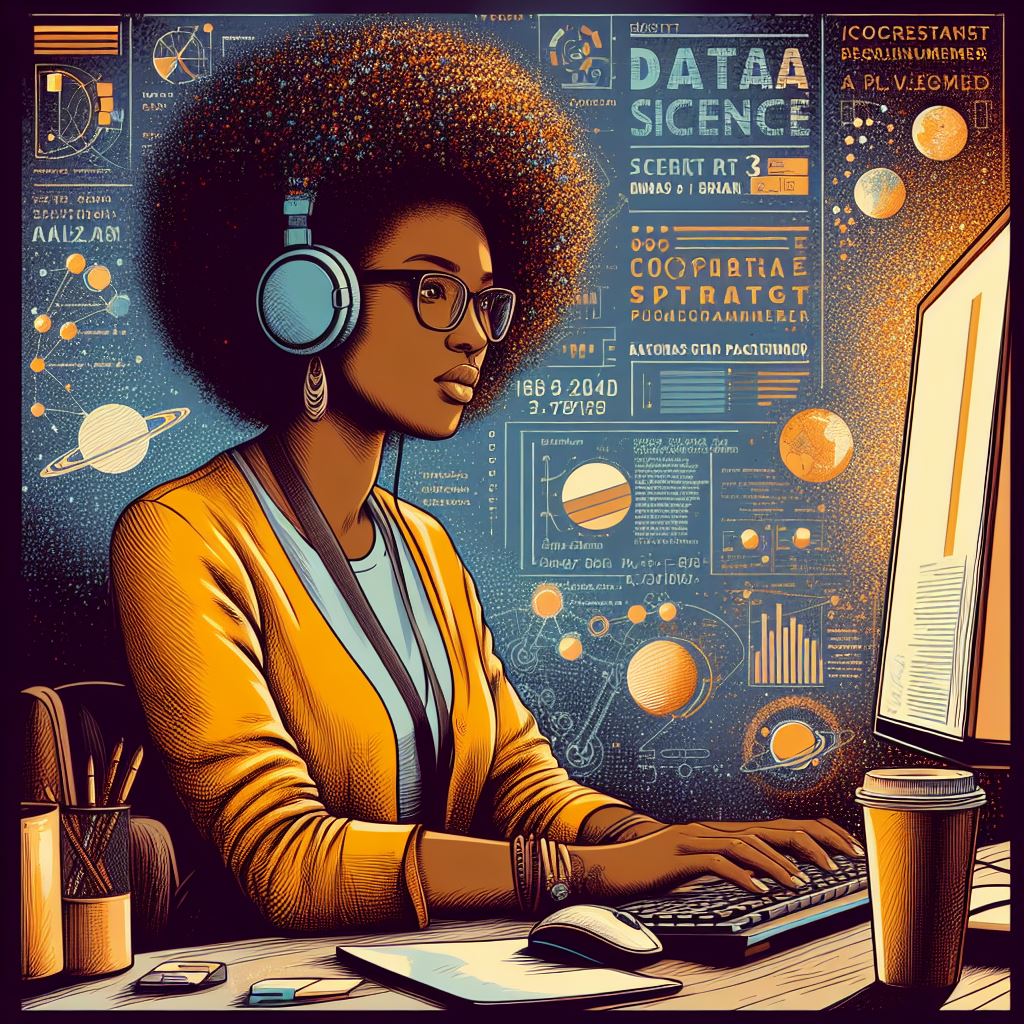