In recent years, machine learning (ML) has emerged as a transformative force in the healthcare sector. By enabling systems to analyze vast amounts of data, ML applications are improving patient care, streamlining medical processes, and driving breakthroughs in medical research. With its potential to diagnose diseases, predict outcomes, and personalize treatment plans, machine learning is changing how healthcare providers deliver services, making them more efficient and effective.
One of the primary ML applications in healthcare is predictive analytics. Machine learning algorithms can analyze patient data, including medical histories, test results, and genetic information, to predict the likelihood of diseases such as cancer, diabetes, or heart conditions. This enables early intervention, which is crucial for improving patient outcomes and reducing treatment costs. ML models can detect patterns and correlations within large datasets that might be too complex for traditional analytical methods.
In addition to predictive capabilities, ML is playing a significant role in diagnostics. For instance, ML-powered tools are used in radiology to interpret medical images like X-rays, MRIs, and CT scans. These tools can detect anomalies, such as tumors or fractures, with high accuracy, assisting radiologists in providing faster and more accurate diagnoses. Similarly, ML models are being used in pathology to analyze tissue samples and identify potential signs of cancer, enabling earlier detection and more targeted treatment options.
Another key area where machine learning is making an impact is in personalized medicine. ML algorithms can analyze genetic data and tailor treatments to individual patients based on their unique genetic profiles. This approach ensures that patients receive the most effective treatments with fewer side effects, ultimately improving overall health outcomes. Personalized medicine is particularly important in oncology, where treatments are increasingly being customized to the genetic characteristics of both the patient and the cancer.
Healthcare organizations are also using ML to optimize operational efficiency. For example, predictive models can help hospitals manage patient flow, reduce wait times, and predict staffing needs. By anticipating demand, hospitals can ensure they have the right resources in place, reducing the likelihood of overburdened staff and ensuring patients receive timely care.
Additionally, ML is transforming drug discovery and development. Traditionally, developing new drugs is a lengthy and expensive process. However, machine learning algorithms can analyze vast datasets to identify potential drug candidates more quickly and accurately. By simulating how different compounds interact with biological systems, ML can help researchers identify promising drug candidates, reducing the time and cost associated with clinical trials.
The use of ML in healthcare is not without challenges. Issues such as data privacy, model transparency, and the need for high-quality training data must be addressed to fully realize the potential of ML in healthcare. Ensuring the ethical use of these technologies and maintaining patient trust is critical as machine learning becomes more integrated into healthcare systems.
In conclusion, machine learning is poised to continue revolutionizing healthcare in numerous ways, from improving diagnosis accuracy to enabling personalized treatment plans and optimizing healthcare operations. As technology continues to advance, the role of ML in healthcare will expand, creating new opportunities for enhancing patient care and medical research. Healthcare providers, researchers, and policymakers must work together to address challenges while embracing the transformative potential of machine learning in healthcare.
5
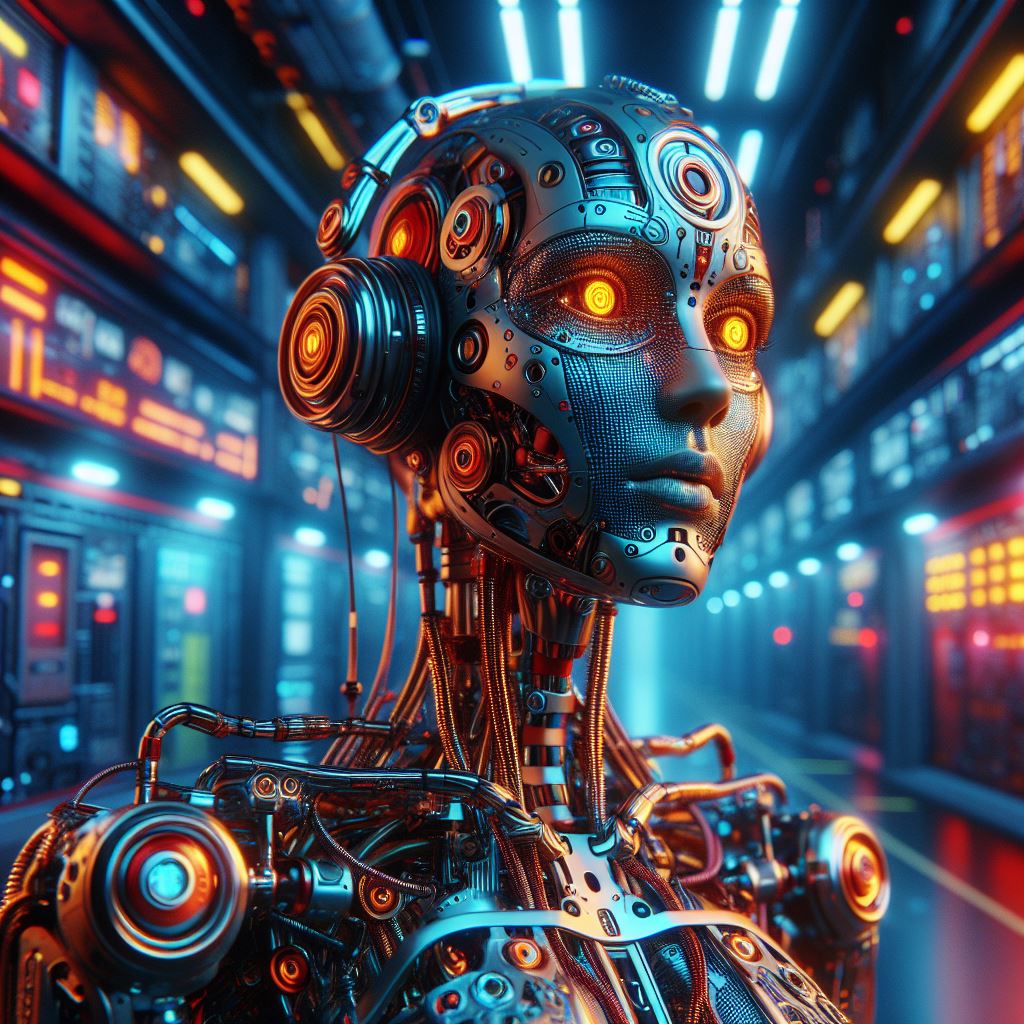