Machine learning (ML) has made significant strides in various industries, and the healthcare sector is no exception. With the ability to analyze vast amounts of data and recognize complex patterns, ML is transforming patient care, enhancing operational efficiency, and improving treatment outcomes. In this article, we will explore the most prominent healthcare ML applications and how they are shaping the future of healthcare.
Predictive Analytics for Patient Health
Machine learning models are increasingly being used for predictive analytics in healthcare. By analyzing historical patient data, these models can predict potential health risks, disease outbreaks, and patient conditions before they become critical. This can lead to early intervention, reducing hospital readmission rates, and potentially saving lives. For example, ML models are used to predict the likelihood of a patient developing conditions like diabetes, cardiovascular diseases, or sepsis, allowing for timely preventive measures.
Personalized Treatment Plans
Another significant application of machine learning in healthcare is the development of personalized treatment plans. Traditionally, treatment methods are standardized, but ML enables the customization of treatments based on individual patient data. By evaluating a patient’s genetic information, medical history, lifestyle, and other relevant factors, ML algorithms can suggest the most effective treatment options. This helps healthcare providers deliver more precise and efficient care, improving patient outcomes.
Medical Imaging and Diagnostics
ML has revolutionized medical imaging, enabling faster and more accurate diagnostics. Using algorithms trained on vast datasets of medical images, ML models can assist in identifying conditions such as tumors, fractures, and other abnormalities with remarkable accuracy. For example, ML is commonly used in radiology for detecting signs of cancer in mammograms, X-rays, and MRI scans. These systems can help radiologists make quicker, more accurate diagnoses, ultimately improving treatment outcomes.
Drug Discovery and Development
The drug discovery process has traditionally been time-consuming and costly. However, ML is speeding up this process by predicting how different compounds will interact with biological systems. Using large datasets and advanced algorithms, researchers can identify promising drug candidates faster and more accurately. ML models are already being used to find new treatments for diseases like cancer, Alzheimer’s, and COVID-19, potentially revolutionizing the way drugs are developed and brought to market.
Operational Efficiency in Healthcare Facilities
In addition to patient care, machine learning is enhancing the operational efficiency of healthcare facilities. ML algorithms can optimize hospital scheduling, predict patient flow, and manage inventory. These applications help reduce wait times, improve resource allocation, and ensure that medical professionals can focus on what matters most—patient care. For example, ML can predict patient admission rates, helping hospitals plan staff schedules and reduce over-crowding.
Conclusion:
The integration of machine learning into healthcare has proven to be a game-changer. From improving diagnostic accuracy to optimizing treatment plans and drug development, ML is paving the way for a more efficient, personalized, and effective healthcare system. As these technologies continue to evolve, healthcare professionals and institutions must stay ahead of the curve to fully harness the potential of machine learning and improve patient outcomes.
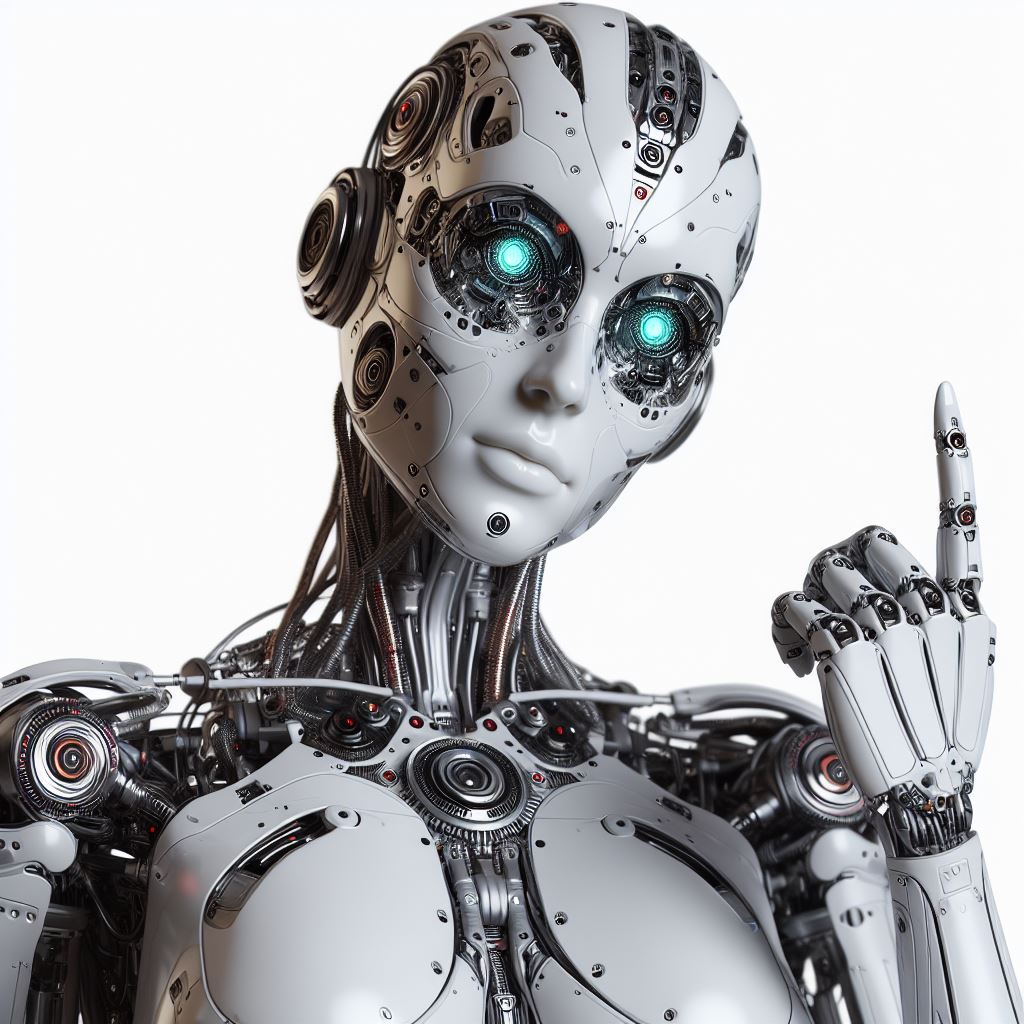