In recent years, the integration of reinforcement learning (RL) in robotics has paved the way for groundbreaking innovations in various industries, with agriculture being a prime beneficiary. Reinforcement learning, a branch of machine learning, focuses on training agents to make decisions by interacting with their environment and receiving feedback based on their actions. This ability to improve through trial and error has led to significant advancements in robotics, making farming processes more efficient and sustainable.
Robotic systems powered by reinforcement learning have become increasingly adept at automating tasks that were once labor-intensive and time-consuming in agriculture. From planting and harvesting crops to monitoring plant health and soil quality, RL-powered robots can enhance precision and productivity. These robots can learn optimal strategies for carrying out tasks such as seed placement, irrigation, and crop harvesting, reducing the need for human intervention and ensuring greater consistency in performance.
In agriculture, tasks like weeding, crop inspection, and pest control often require constant attention and labor, and automation through robotics can address these challenges. RL algorithms enable robots to learn how to navigate complex and ever-changing farm environments, adapting to variables such as weather conditions, soil types, and plant growth stages. For instance, RL can help a robot adjust its approach to irrigation depending on soil moisture levels, or determine the most efficient route to harvest crops without damaging them.
One of the most exciting aspects of RL in agriculture is its ability to contribute to sustainability. Traditional farming practices often lead to overuse of resources such as water and fertilizers. By utilizing reinforcement learning, robots can optimize resource usage, reducing waste and minimizing the environmental impact of farming. For example, RL can guide robots to apply fertilizers or pesticides only when and where they are needed, minimizing chemical use and preserving soil health.
Another significant advantage of RL in agricultural robotics is scalability. As farm sizes grow and the need for more efficient farming techniques becomes greater, RL-based systems can be expanded to handle larger, more diverse operations. These systems can learn from data collected across multiple farms, adapting and improving based on a wider range of scenarios and conditions. Additionally, RL robots can collaborate with other robots and systems on the farm, optimizing their collective efforts for maximum efficiency.
Furthermore, the use of RL in robotics is transforming the way farmers make decisions. By equipping agricultural robots with the ability to learn and adapt, farmers are empowered to make more informed choices about crop management, resource allocation, and scheduling. With the assistance of RL-powered robots, farmers can focus on strategic decision-making, while the robots handle the execution of routine tasks, freeing up time for more critical activities.
As the field of reinforcement learning continues to evolve, the potential applications in robotics and agriculture are vast. With ongoing advancements in AI and machine learning, the future of farming is increasingly dependent on the collaboration between humans and robots, creating a more efficient, sustainable, and productive agricultural landscape.
5
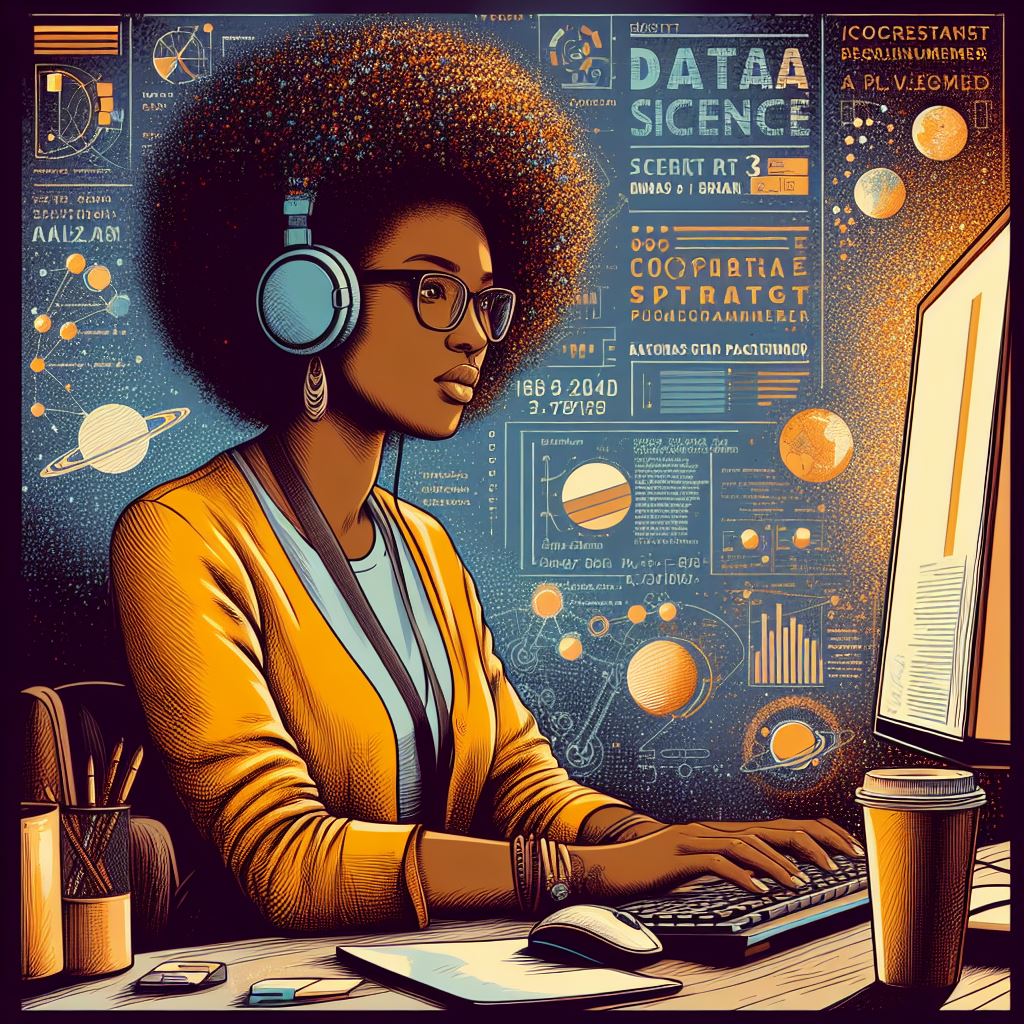