The healthcare industry has seen transformative changes over the past decade, and one of the most notable innovations is the integration of artificial intelligence (AI) into medical imaging. AI technologies, particularly deep learning algorithms, have made significant advancements in improving diagnostic accuracy, efficiency, and patient outcomes. By analyzing medical images such as X-rays, CT scans, MRIs, and ultrasounds, AI is helping healthcare professionals detect diseases earlier and more accurately, which leads to better treatment decisions and ultimately saves lives.
How AI is Enhancing Medical Imaging
Medical imaging has always been a cornerstone of diagnosing diseases and monitoring patient health. Traditionally, the interpretation of these images was done manually by radiologists, but this process is time-consuming and prone to human error. With the advent of AI, these challenges are being addressed by automating image interpretation, allowing for faster and more reliable results.
AI algorithms are trained to recognize patterns in medical images and can identify subtle abnormalities that might be missed by the human eye. For example, AI-powered tools can detect early signs of conditions like cancer, brain disorders, and heart diseases, often before symptoms appear, enabling earlier interventions. This early detection is critical for improving patient survival rates and minimizing the need for invasive treatments.
Key AI Technologies in Medical Imaging
Deep Learning (DL): Deep learning is a subset of AI that uses artificial neural networks to analyze large volumes of data. In medical imaging, deep learning models are trained on thousands of annotated images to learn features and patterns associated with various diseases. These models are capable of recognizing abnormalities such as tumors, fractures, and other medical conditions with high accuracy.
Convolutional Neural Networks (CNNs): CNNs are a type of deep learning algorithm that excels in image processing. In medical imaging, CNNs are used to identify specific features within images like lesions, masses, or calcifications, which are often indicative of serious health issues. CNNs have proven to be highly effective in analyzing complex medical images and delivering accurate diagnoses.
Natural Language Processing (NLP): While NLP is commonly associated with text analysis, it also plays a role in medical imaging. By converting radiology reports into structured data, NLP can be used to track patient histories, enhance image interpretation, and even assist in identifying trends that might suggest a particular diagnosis.
AI-Powered Image Reconstruction: AI is also making strides in the realm of image reconstruction. By improving the quality of images obtained from diagnostic tools, AI can help reduce the time required for scans and ensure better visual clarity, which is critical for accurate diagnosis.
Predictive Analytics: AI technologies in medical imaging also leverage predictive analytics to forecast future health risks. By analyzing past medical images and patient data, AI can help predict the likelihood of developing certain conditions, allowing for preventive measures to be taken in advance.
Benefits of AI in Medical Imaging
AI’s role in medical imaging is not just about improving diagnostic accuracy; it also offers numerous other benefits for the healthcare industry:
Increased Efficiency: AI reduces the workload on radiologists by automating routine tasks like image analysis, enabling them to focus on more complex cases.
Cost-Effective: AI-driven systems can help reduce the cost of healthcare by improving diagnostic workflows and reducing the need for repeat imaging.
Accessibility: AI tools can bring advanced diagnostic capabilities to remote areas where access to specialized healthcare professionals may be limited, thus bridging healthcare gaps.
Challenges and Future Prospects
While AI in medical imaging holds immense promise, there are challenges that must be addressed. The quality of AI predictions depends on the quality of the data used to train the algorithms, and there is a need for large, diverse datasets to ensure generalization across different populations. Additionally, regulatory and ethical concerns must be considered to ensure the responsible use of AI in healthcare.
Despite these challenges, the future of AI in medical imaging looks bright. With continuous advancements in AI technology, medical imaging is poised to become even more precise, efficient, and accessible, paving the way for better healthcare outcomes globally.
5
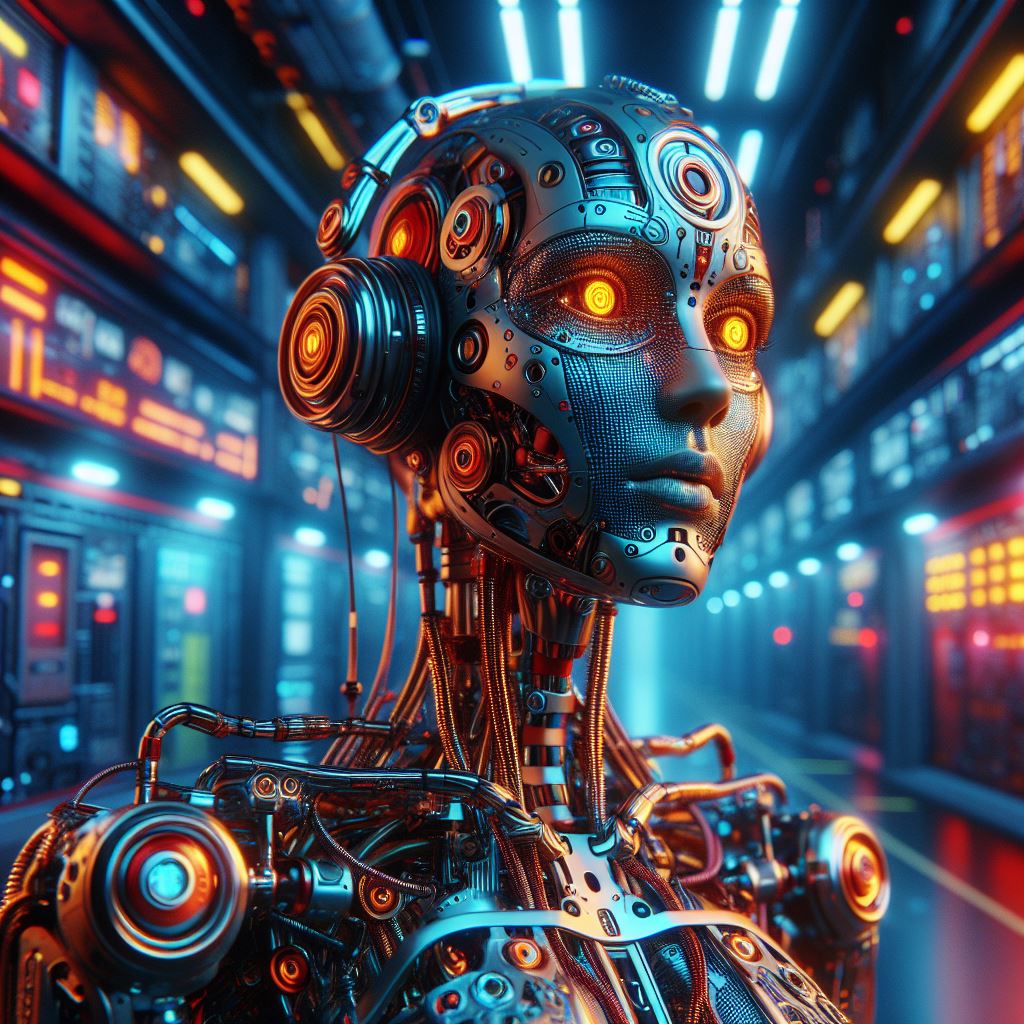