In recent years, artificial intelligence (AI) has made impressive strides in improving how businesses process data and interact with customers. Two cutting-edge technologies leading this transformation are Retrieval-Augmented Generation (RAG) and Large Language Models (LLMs). These innovations, when integrated, are paving the way for more efficient and personalized services across various industries.
What is Retrieval-Augmented Generation (RAG)?
Retrieval-Augmented Generation (RAG) is a method used to enhance the capabilities of language models. It combines the power of retrieving information from large document databases with the generative abilities of AI models. Traditional language models generate responses based on the training data they’ve absorbed, but they can often struggle when asked about topics not seen during their training.
RAG overcomes this limitation by incorporating a retrieval mechanism that allows the AI to search and pull relevant documents from external sources before generating its response. This ensures that the AI model can provide more accurate and contextually relevant information, even when dealing with specialized or niche queries. In business, this can significantly improve customer service, decision-making, and data analysis by allowing the AI to access the most up-to-date and relevant content available.
The Role of Large Language Models (LLMs) in RAG
Large Language Models (LLMs), such as OpenAI’s GPT-3 or GPT-4, are AI models trained on vast amounts of text data. They have the ability to understand and generate human-like text, making them valuable for tasks like content creation, language translation, and natural language processing (NLP).
When combined with RAG, LLMs become even more powerful. The retrieval mechanism ensures that the LLM has access to a broader set of documents and information, enabling it to generate highly accurate and context-aware responses. For example, in a customer support application, an LLM integrated with RAG can pull up relevant company manuals, FAQs, or external resources, ensuring that the AI’s response is not only linguistically coherent but also factually correct and up-to-date.
How RAG and LLMs Are Transforming Business Operations
Customer Support Automation: RAG combined with LLMs allows businesses to automate customer support at a higher level of accuracy. AI can retrieve context-specific information and generate personalized responses to customers, reducing the workload on human agents and improving customer satisfaction.
Content Creation and Marketing: Businesses can leverage RAG and LLMs for content creation by retrieving market insights, relevant articles, and research to generate high-quality blog posts, reports, and marketing materials. This ensures the content is both relevant and informed, driving engagement with target audiences.
Data Analysis and Decision Making: RAG’s ability to retrieve up-to-date data combined with LLMs’ generative capabilities can significantly enhance business decision-making. AI can process and synthesize large amounts of data, providing actionable insights and helping companies stay ahead of market trends.
Training and Knowledge Management: Organizations can build knowledge repositories that RAG-powered systems can query, allowing employees to quickly retrieve training materials and information. This helps to maintain a consistent knowledge base and enhances employee productivity and learning.
The Future of RAG and LLMs in Business
As the integration of RAG and LLM technologies continues to evolve, businesses will see more sophisticated applications in areas like healthcare, finance, and education. AI models will become even more adept at combining large datasets with real-time information, leading to smarter, more efficient processes. Furthermore, advancements in AI transparency and ethical guidelines will ensure that RAG and LLM systems are used responsibly, fostering trust in AI-powered solutions.
Businesses that embrace RAG and LLMs will be better equipped to stay competitive in an increasingly data-driven world. By automating complex tasks, improving decision-making processes, and providing enhanced customer experiences, these technologies offer a roadmap for sustainable growth and innovation.
5
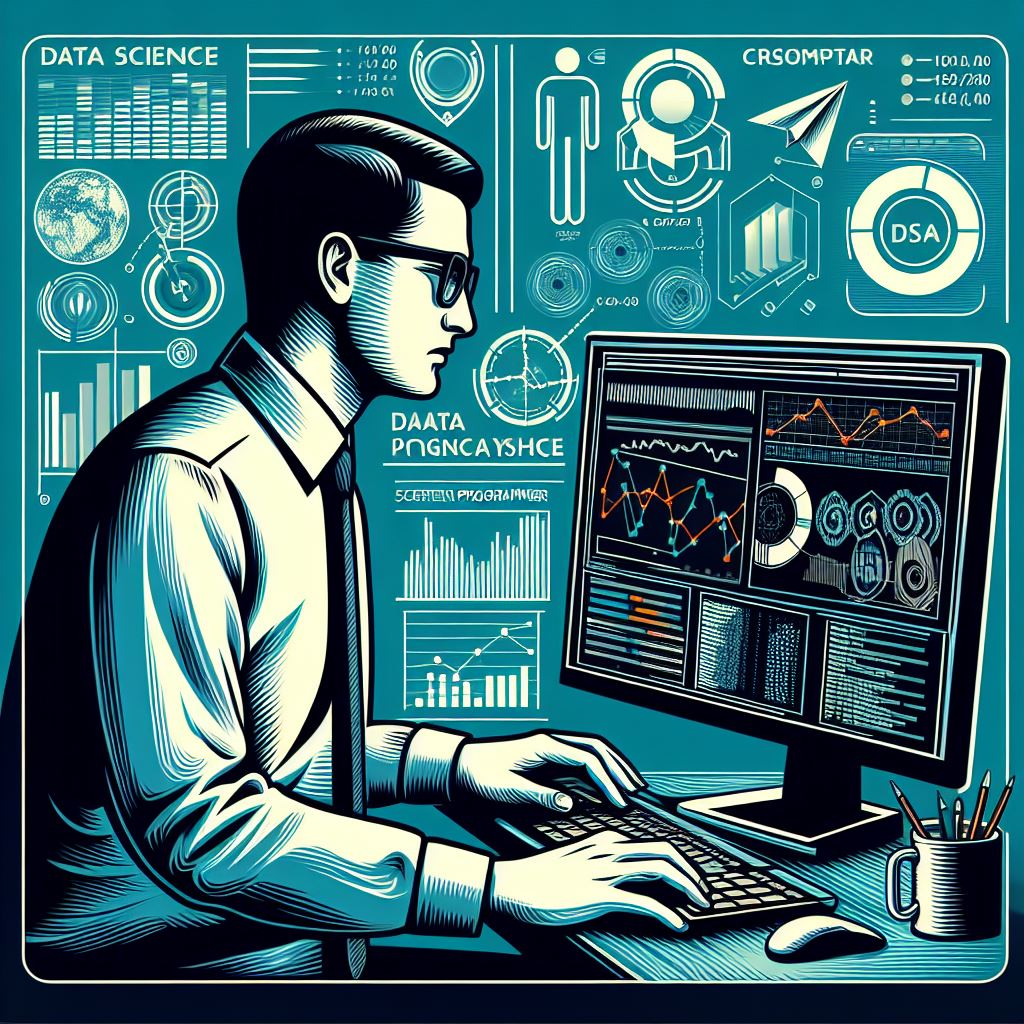