Reinforcement learning (RL) has emerged as a groundbreaking technique in the field of artificial intelligence (AI), and its applications in robotics have transformed industries like agriculture. In recent years, combining reinforcement learning with robotics has led to significant advancements in automating agricultural processes, improving efficiency, and optimizing resource usage. By leveraging the power of RL, robots are learning how to make decisions based on environmental feedback, ultimately benefiting farmers and the agricultural sector.
Understanding Reinforcement Learning
Reinforcement learning is a type of machine learning where an agent learns how to behave in an environment by performing actions and receiving feedback through rewards or penalties. The agent seeks to maximize its cumulative reward, which drives it to explore various strategies for optimal decision-making. In agriculture, reinforcement learning algorithms enable robots to adapt to dynamic environments, such as unpredictable weather conditions or varying crop types, by continuously learning and improving their performance.
The Impact of Robotics on Agriculture
Robotics in agriculture has seen rapid adoption due to its ability to automate labor-intensive tasks. Robots equipped with AI, such as autonomous tractors, drones, and harvesting machines, can perform various functions like planting seeds, spraying pesticides, and collecting data. However, the real potential lies in integrating reinforcement learning, which empowers these robots to operate autonomously, make real-time decisions, and continuously optimize their actions for better results.
For example, autonomous tractors equipped with RL algorithms can optimize their paths for planting crops by learning the most efficient routes based on terrain and soil conditions. This not only reduces fuel consumption but also ensures consistent crop growth by minimizing the impact of human error and optimizing field management strategies.
RL Applications in Agricultural Robotics
Reinforcement learning is being used in agricultural robotics to address several challenges:
Autonomous Crop Monitoring: Drones and ground robots can use RL to monitor crop health by learning to identify patterns in plant growth, disease, or pests. These robots can autonomously navigate fields, detect anomalies, and recommend or even perform corrective actions, such as applying fertilizers or pesticides only where needed, reducing waste and environmental impact.
Precision Agriculture: In precision agriculture, RL helps robots learn the optimal distribution of water, fertilizers, and pesticides. By analyzing data from various sensors in real-time, robots can make adjustments based on factors such as weather, soil moisture, and crop health. This leads to higher yields, reduced resource wastage, and cost savings for farmers.
Weeding and Harvesting: Robots equipped with RL can learn to differentiate between crops and weeds, autonomously removing weeds without damaging valuable plants. In harvesting, RL algorithms help robots decide the best time and method to harvest crops, ensuring optimal yield and quality.
Resource Management: With RL, agricultural robots can manage resources such as water and fertilizers more effectively. By learning how different environmental conditions affect crop growth, robots can predict and optimize the amount of water and nutrients needed for each specific area of a field.
Challenges and Future Prospects
While reinforcement learning offers immense potential in agricultural robotics, there are still challenges to overcome. The primary challenge is the complexity of real-world environments, which require robots to deal with uncertainty, variable conditions, and a wide range of tasks. Additionally, training RL agents for agriculture requires large amounts of high-quality data and computational resources.
However, the future of RL in agriculture looks promising. As the technology continues to evolve, we can expect to see even more autonomous robots working alongside farmers to optimize every aspect of crop production, from planting to harvesting. With RL-driven robots, the agricultural sector can become more sustainable, efficient, and resilient to the challenges posed by climate change and a growing global population.
Conclusion
Reinforcement learning in robotics is revolutionizing agriculture by enabling autonomous systems that can make intelligent decisions based on real-time data and environmental feedback. From crop monitoring to resource management, RL-driven robots are helping farmers increase productivity while reducing waste and costs. As the technology matures, the integration of RL with robotics will play a pivotal role in shaping the future of agriculture.
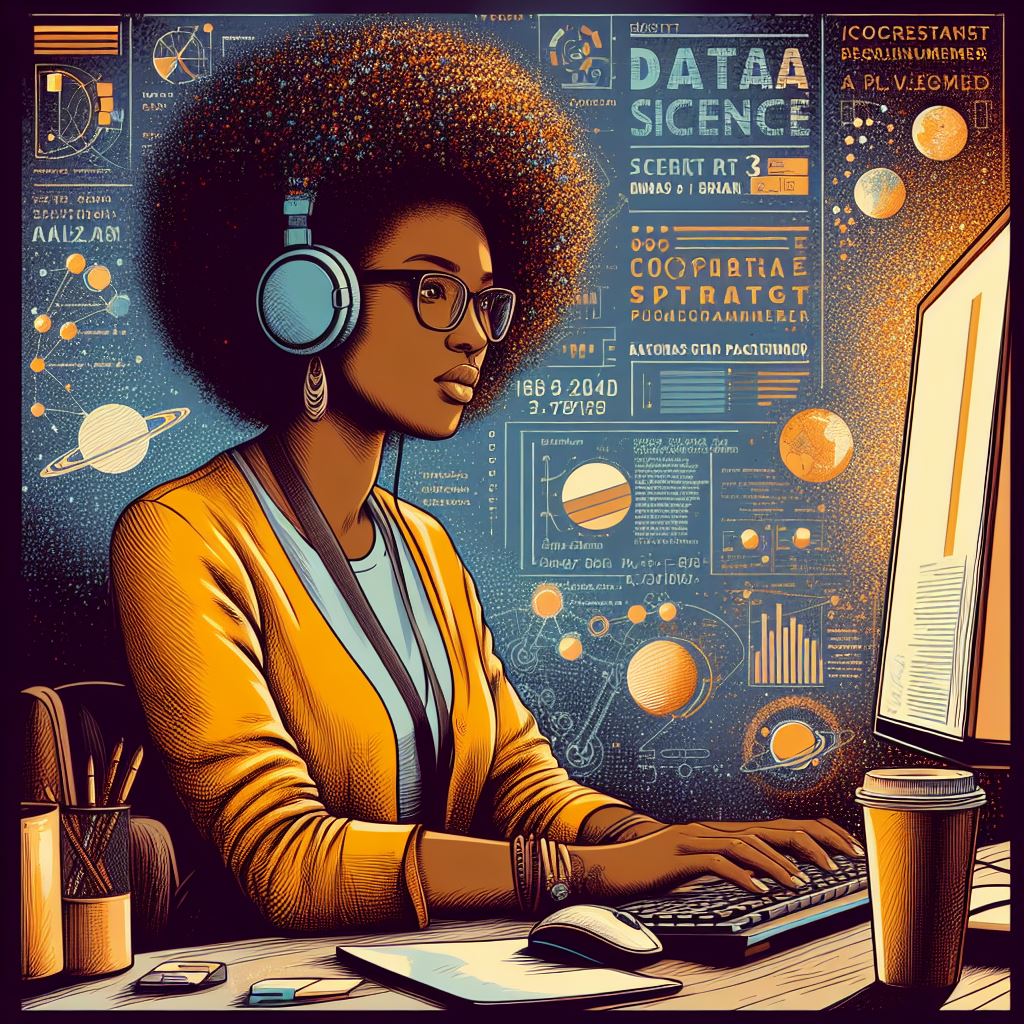